arXiv:2501.09898v1 Announce Type: new
Abstract: Tremendous progress has been made in deep stereo matching to excel on benchmark datasets through per-domain fine-tuning. However, achieving strong zero-shot generalization – a hallmark of foundation models in other computer vision tasks – remains challenging for stereo matching. We introduce FoundationStereo, a foundation model for stereo depth estimation designed to achieve strong zero-shot generalization. To this end, we first construct a large-scale (1M stereo pairs) synthetic training dataset featuring large diversity and high photorealism, followed by an automatic self-curation pipeline to remove ambiguous samples. We then design a number of network architecture components to enhance scalability, including a side-tuning feature backbone that adapts rich monocular priors from vision foundation models to mitigate the sim-to-real gap, and long-range context reasoning for effective cost volume filtering. Together, these components lead to strong robustness and accuracy across domains, establishing a new standard in zero-shot stereo depth estimation.
Source link
lol
FoundationStereo: Zero-Shot Stereo Matching
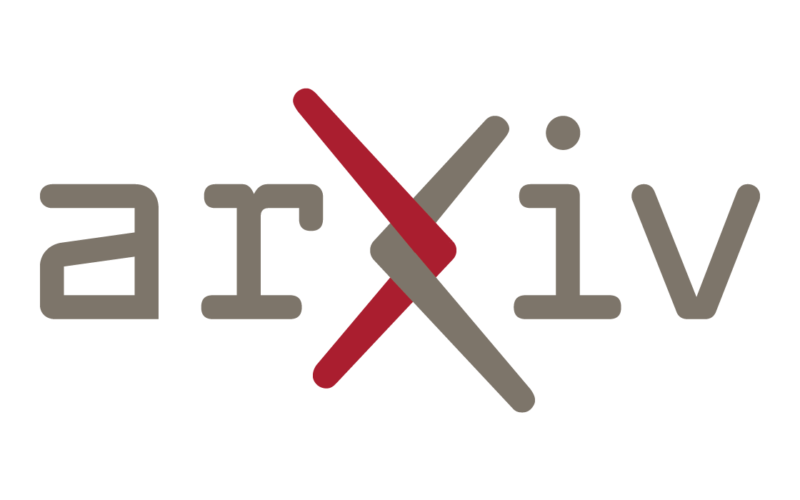