arXiv:2501.09851v1 Announce Type: new
Abstract: We study the problem of PAC learning $gamma$-margin halfspaces with Massart noise. We propose a simple proper learning algorithm, the Perspectron, that has sample complexity $widetilde{O}((epsilongamma)^{-2})$ and achieves classification error at most $eta+epsilon$ where $eta$ is the Massart noise rate. Prior works [DGT19,CKMY20] came with worse sample complexity guarantees (in both $epsilon$ and $gamma$) or could only handle random classification noise [DDK+23,KIT+23] — a much milder noise assumption. We also show that our results extend to the more challenging setting of learning generalized linear models with a known link function under Massart noise, achieving a similar sample complexity to the halfspace case. This significantly improves upon the prior state-of-the-art in this setting due to [CKMY20], who introduced this model.
Source link
lol
Learning Noisy Halfspaces with a Margin: Massart is No Harder than Random
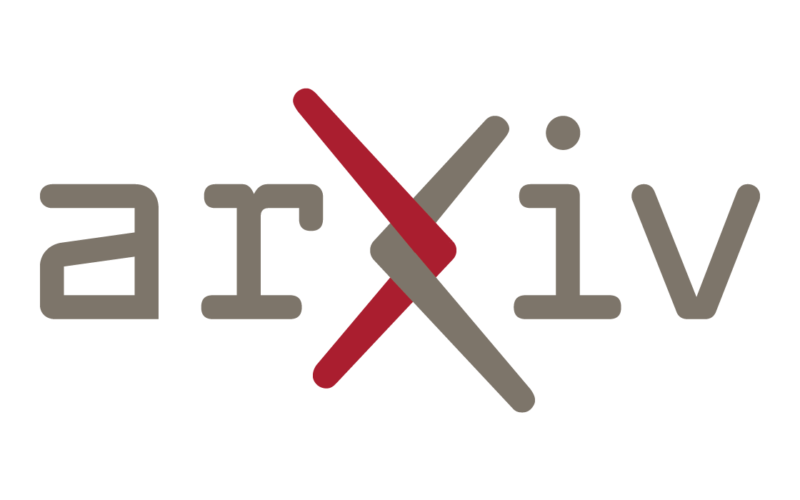