arXiv:2501.09878v1 Announce Type: new
Abstract: We present ASTRA (A} Scene-aware TRAnsformer-based model for trajectory prediction), a light-weight pedestrian trajectory forecasting model that integrates the scene context, spatial dynamics, social inter-agent interactions and temporal progressions for precise forecasting. We utilised a U-Net-based feature extractor, via its latent vector representation, to capture scene representations and a graph-aware transformer encoder for capturing social interactions. These components are integrated to learn an agent-scene aware embedding, enabling the model to learn spatial dynamics and forecast the future trajectory of pedestrians. The model is designed to produce both deterministic and stochastic outcomes, with the stochastic predictions being generated by incorporating a Conditional Variational Auto-Encoder (CVAE). ASTRA also proposes a simple yet effective weighted penalty loss function, which helps to yield predictions that outperform a wide array of state-of-the-art deterministic and generative models. ASTRA demonstrates an average improvement of 27%/10% in deterministic/stochastic settings on the ETH-UCY dataset, and 26% improvement on the PIE dataset, respectively, along with seven times fewer parameters than the existing state-of-the-art model (see Figure 1). Additionally, the model’s versatility allows it to generalize across different perspectives, such as Bird’s Eye View (BEV) and Ego-Vehicle View (EVV).
Source link
lol
ASTRA: A Scene-aware TRAnsformer-based model for trajectory prediction
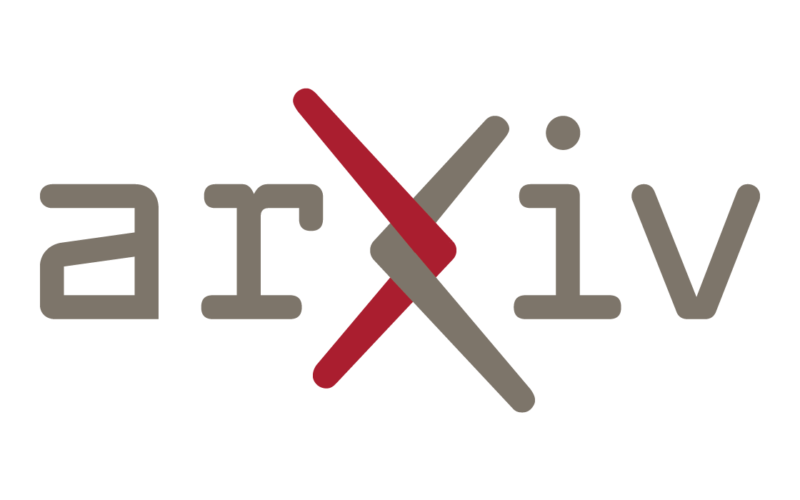