arXiv:2501.08538v1 Announce Type: new
Abstract: Heterogeneous graph pre-training (HGP) has demonstrated remarkable performance across various domains. However, the issue of heterophily in real-world heterogeneous graphs (HGs) has been largely overlooked. To bridge this research gap, we proposed a novel heterogeneous graph contrastive learning framework, termed HGMS, which leverages connection strength and multi-view self-expression to learn homophilous node representations. Specifically, we design a heterogeneous edge dropping augmentation strategy that enhances the homophily of augmented views. Moreover, we introduce a multi-view self-expressive learning method to infer the homophily between nodes. In practice, we develop two approaches to solve the self-expressive matrix. The solved self-expressive matrix serves as an additional augmented view to provide homophilous information and is used to identify false negatives in contrastive loss. Extensive experimental results demonstrate the superiority of HGMS across different downstream tasks.
Source link
lol
Homophily-aware Heterogeneous Graph Contrastive Learning
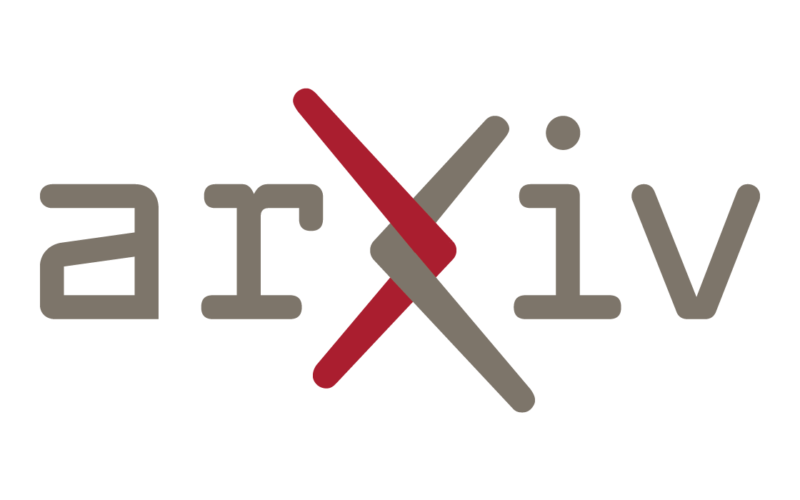