arXiv:2501.08468v1 Announce Type: new
Abstract: While Speech Foundation Models (SFMs) excel in various speech tasks, their performance for low-resource tasks such as child Automatic Speech Recognition (ASR) is hampered by limited pretraining data. To address this, we explore different model merging techniques to leverage knowledge from models trained on larger, more diverse speech corpora. This paper also introduces Selective Attention (SA) Merge, a novel method that selectively merges task vectors from attention matrices to enhance SFM performance on low-resource tasks. Experiments on the MyST database show significant reductions in relative word error rate of up to 14%, outperforming existing model merging and data augmentation techniques. By combining data augmentation techniques with SA Merge, we achieve a new state-of-the-art WER of 8.69 on the MyST database for the Whisper-small model, highlighting the potential of SA Merge for improving low-resource ASR.
Source link
lol
Selective Attention Merging for low resource tasks: A case study of Child ASR
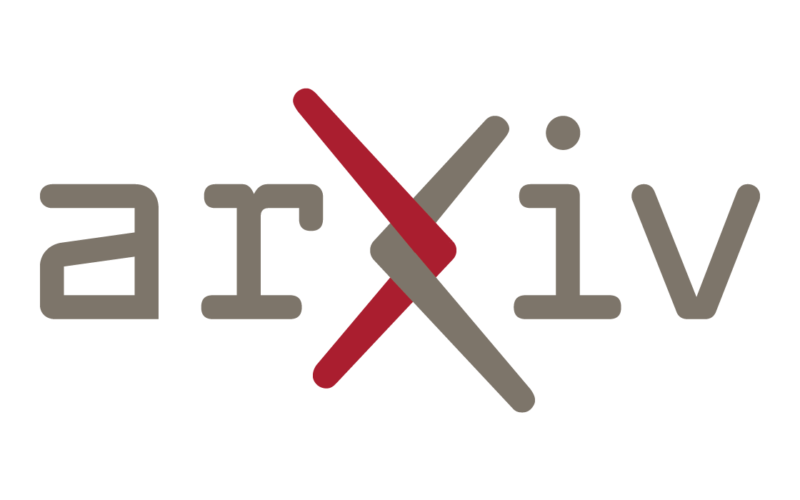