arXiv:2501.07972v1 Announce Type: cross
Abstract: The target of video moment retrieval (VMR) is predicting temporal spans within a video that semantically match a given linguistic query. Existing VMR methods based on multimodal large language models (MLLMs) overly rely on expensive high-quality datasets and time-consuming fine-tuning. Although some recent studies introduce a zero-shot setting to avoid fine-tuning, they overlook inherent language bias in the query, leading to erroneous localization. To tackle the aforementioned challenges, this paper proposes Moment-GPT, a tuning-free pipeline for zero-shot VMR utilizing frozen MLLMs. Specifically, we first employ LLaMA-3 to correct and rephrase the query to mitigate language bias. Subsequently, we design a span generator combined with MiniGPT-v2 to produce candidate spans adaptively. Finally, to leverage the video comprehension capabilities of MLLMs, we apply VideoChatGPT and span scorer to select the most appropriate spans. Our proposed method substantially outperforms the state-ofthe-art MLLM-based and zero-shot models on several public datasets, including QVHighlights, ActivityNet-Captions, and Charades-STA.
Source link
lol
Zero-shot Video Moment Retrieval via Off-the-shelf Multimodal Large Language Models
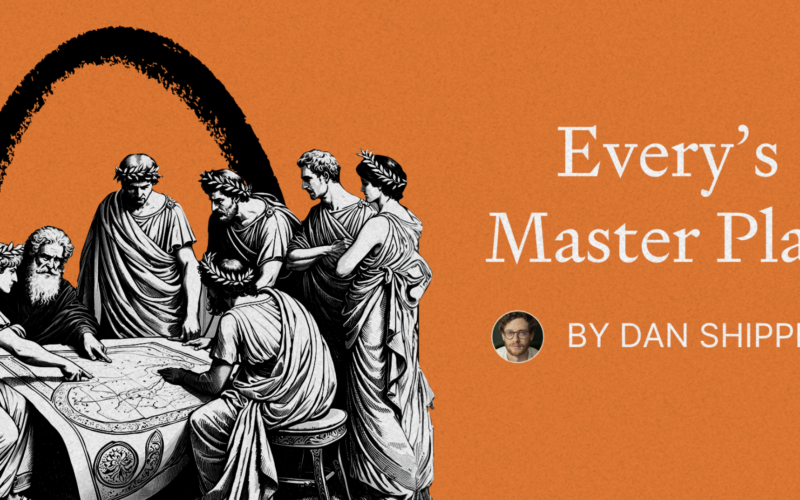