arXiv:2501.06238v1 Announce Type: new
Abstract: Feature level sets (FLS) have shown significant potential in the analysis of multi-field data by using traits defined in attribute space to specify features in the domain. In this work, we address key challenges in the practical use of FLS: trait design and feature selection for rendering. To simplify trait design, we propose a Cartesian decomposition of traits into simpler components, making the process more intuitive and computationally efficient. Additionally, we utilize dictionary learning results to automatically suggest point traits. To enhance feature selection, we introduce trait-induced merge trees (TIMTs), a generalization of merge trees for feature level sets, aimed at topologically analyzing tensor fields or general multi-variate data. The leaves in the TIMT represent areas in the input data that are closest to the defined trait, thereby most closely resembling the defined feature. This merge tree provides a hierarchy of features, enabling the querying of the most relevant and persistent features. Our method includes various query techniques for the tree, allowing the highlighting of different aspects. We demonstrate the cross-application capabilities of this approach through five case studies from different domains.
Source link
lol
Multi-field Visualization: Trait design and trait-induced merge trees
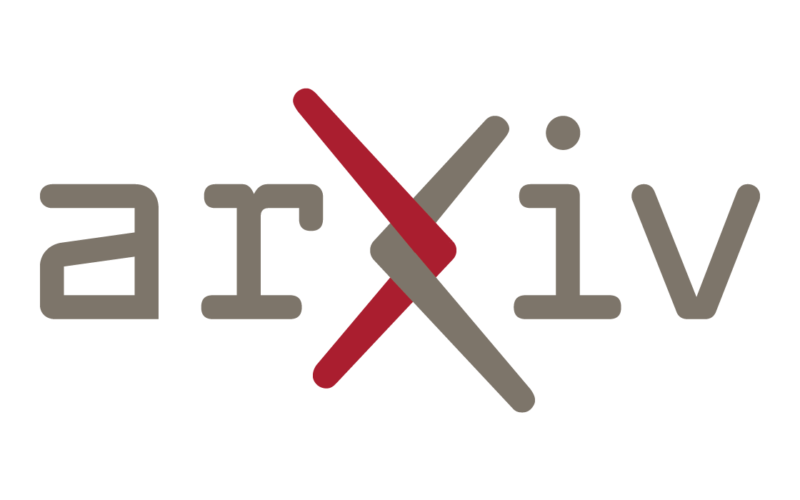