arXiv:2501.06466v1 Announce Type: cross
Abstract: This work introduces a novel application for predicting the macroscopic intrinsic permeability tensor in deformable porous media, using a limited set of micro-CT images of real microgeometries. The primary goal is to develop an efficient, machine-learning (ML)-based method that overcomes the limitations of traditional permeability estimation techniques, which often rely on time-consuming experiments or computationally expensive fluid dynamics simulations. The novelty of this work lies in leveraging Convolutional Neural Networks (CNN) to predict pore-fluid flow behavior under deformation and anisotropic flow conditions. Particularly, the described approach employs binarized CT images of porous micro-structure as inputs to predict the symmetric second-order permeability tensor, a critical parameter in continuum porous media flow modeling. The methodology comprises four key steps: (1) constructing a dataset of CT images from Bentheim sandstone at different volumetric strain levels; (2) performing pore-scale simulations of single-phase flow using the lattice Boltzmann method (LBM) to generate permeability data; (3) training the CNN model with the processed CT images as inputs and permeability tensors as outputs; and (4) exploring techniques to improve model generalization, including data augmentation and alternative CNN architectures. Examples are provided to demonstrate the CNN’s capability to accurately predict the permeability tensor, a crucial parameter in various disciplines such as geotechnical engineering, hydrology, and material science. An exemplary source code is made available for interested readers.
Source link
lol
CNN-powered micro- to macro-scale flow modeling in deformable porous media
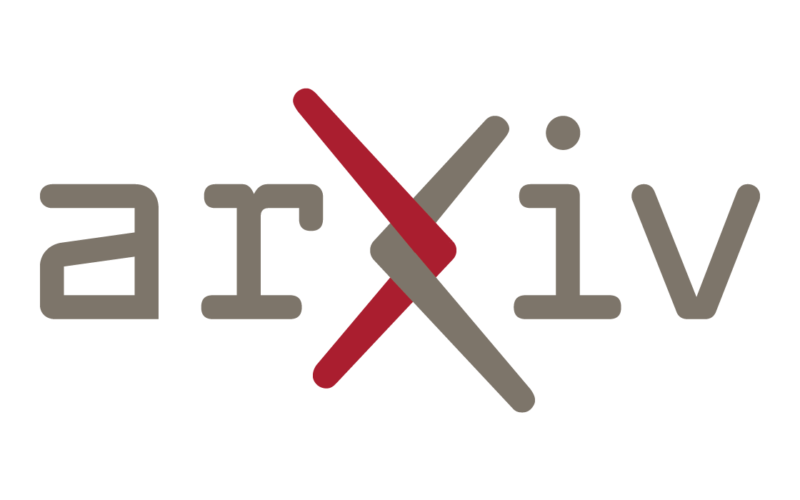