arXiv:2501.04958v1 Announce Type: new
Abstract: Deep learning models in medical imaging face dual challenges: domain shift, where models perform poorly when deployed in settings different from their training environment, and class imbalance, where certain disease conditions are naturally underrepresented. We present Imbalance-Aware Domain Adaptation (IADA), a novel framework that simultaneously tackles both challenges through three key components: (1) adaptive feature learning with class-specific attention mechanisms, (2) balanced domain alignment with dynamic weighting, and (3) adaptive threshold optimization. Our theoretical analysis establishes convergence guarantees and complexity bounds. Through extensive experiments on embryo development assessment across four imaging modalities, IADA demonstrates significant improvements over existing methods, achieving up to 25.19% higher accuracy while maintaining balanced performance across classes. In challenging scenarios with low-quality imaging systems, IADA shows robust generalization with AUC improvements of up to 12.56%. These results demonstrate IADA’s potential for developing reliable and equitable medical imaging systems for diverse clinical settings. The code is made public available at url{https://github.com/yinghemedical/imbalance-aware_domain_adaptation}
Source link
lol
Addressing Domain Shift via Imbalance-Aware Domain Adaptation in Embryo Development Assessment
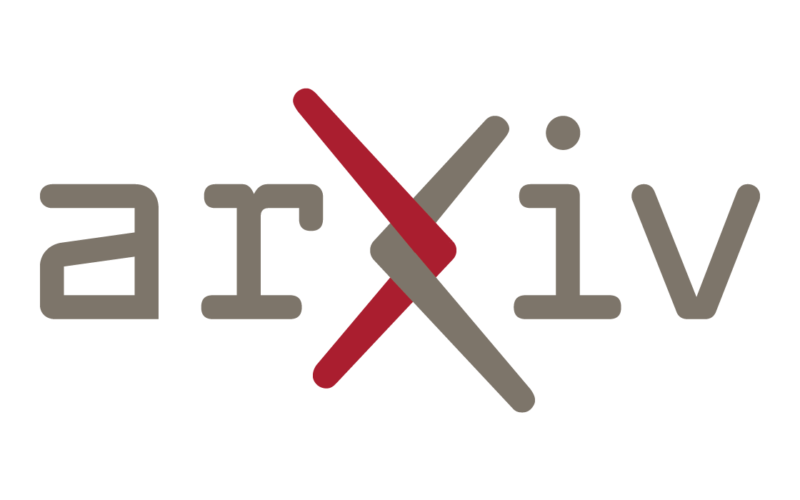