arXiv:2501.04858v1 Announce Type: new
Abstract: This paper examines the specific obstacles of constructing Retrieval-Augmented Generation(RAG) systems in low-resource languages, with a focus on Persian’s complicated morphology and versatile syntax. The research aims to improve retrieval and generation accuracy by introducing Persian-specific models, namely MatinaRoberta(a masked language model) and MatinaSRoberta(a fine-tuned Sentence-BERT), along with a comprehensive benchmarking framework. Three datasets-general knowledge(PQuad), scientifically specialized texts, and organizational reports, were used to assess these models after they were trained on a varied corpus of 73.11 billion Persian tokens. The methodology involved extensive pretraining, fine-tuning with tailored loss functions, and systematic evaluations using both traditional metrics and the Retrieval-Augmented Generation Assessment framework. The results show that MatinaSRoberta outperformed previous embeddings, achieving superior contextual relevance and retrieval accuracy across datasets. Temperature tweaking, chunk size modifications, and document summary indexing were explored to enhance RAG setups. Larger models like Llama-3.1 (70B) consistently demonstrated the highest generation accuracy, while smaller models faced challenges with domain-specific and formal contexts. The findings underscore the potential for developing RAG systems in Persian through customized embeddings and retrieval-generation settings and highlight the enhancement of NLP applications such as search engines and legal document analysis in low-resource languages.
Source link
lol
Advancing Retrieval-Augmented Generation for Persian: Development of Language Models, Comprehensive Benchmarks, and Best Practices for Optimization
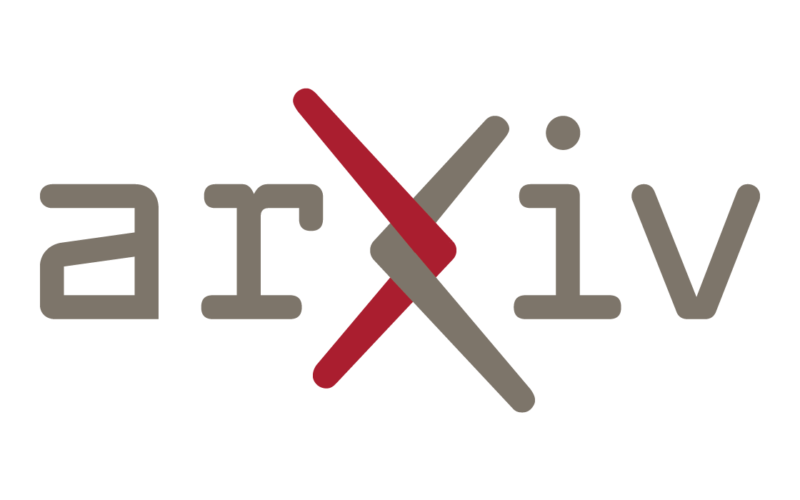