arXiv:2501.03265v1 Announce Type: new
Abstract: The emergence of 5G and edge computing hardware has brought about a significant shift in artificial intelligence, with edge AI becoming a crucial technology for enabling intelligent applications. With the growing amount of data generated and stored on edge devices, deploying AI models for local processing and inference has become increasingly necessary. However, deploying state-of-the-art AI models on resource-constrained edge devices faces significant challenges that must be addressed. This paper presents an optimization triad for efficient and reliable edge AI deployment, including data, model, and system optimization. First, we discuss optimizing data through data cleaning, compression, and augmentation to make it more suitable for edge deployment. Second, we explore model design and compression methods at the model level, such as pruning, quantization, and knowledge distillation. Finally, we introduce system optimization techniques like framework support and hardware acceleration to accelerate edge AI workflows. Based on an in-depth analysis of various application scenarios and deployment challenges of edge AI, this paper proposes an optimization paradigm based on the data-model-system triad to enable a whole set of solutions to effectively transfer ML models, which are initially trained in the cloud, to various edge devices for supporting multiple scenarios.
Source link
lol
Optimizing Edge AI: A Comprehensive Survey on Data, Model, and System Strategies
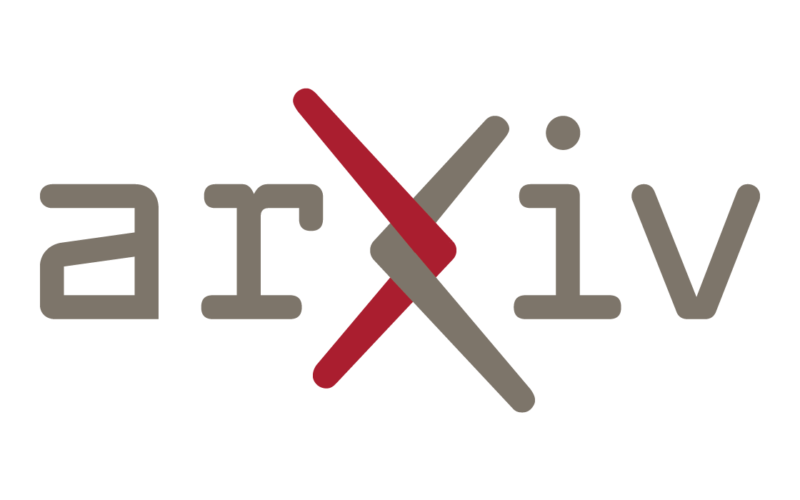