arXiv:2501.03273v1 Announce Type: new
Abstract: This study investigates transformer model compression by systematically pruning its layers. We evaluated 14 pruning strategies across nine diverse datasets, including 12 strategies based on different signals obtained from layer activations, mutual information, gradients, weights, and attention. To address the limitations of single-signal strategies, we introduced two fusion strategies, linear regression and random forest, which combine individual strategies (i.e., strategic fusion), for more informed pruning decisions. Additionally, we applied knowledge distillation to mitigate any accuracy loss during layer pruning. Our results reveal that random forest strategic fusion outperforms individual strategies in seven out of nine datasets and achieves near-optimal performance in the other two. The distilled random forest surpasses the original accuracy in six datasets and mitigates accuracy drops in the remaining three. Knowledge distillation also improves the accuracy-to-size ratio by an average factor of 18.84 across all datasets. Supported by mathematical foundations and biological analogies, our findings suggest that strategically combining multiple signals can lead to efficient, high-performing transformer models for resource-constrained applications.
Source link
lol
Strategic Fusion Optimizes Transformer Compression
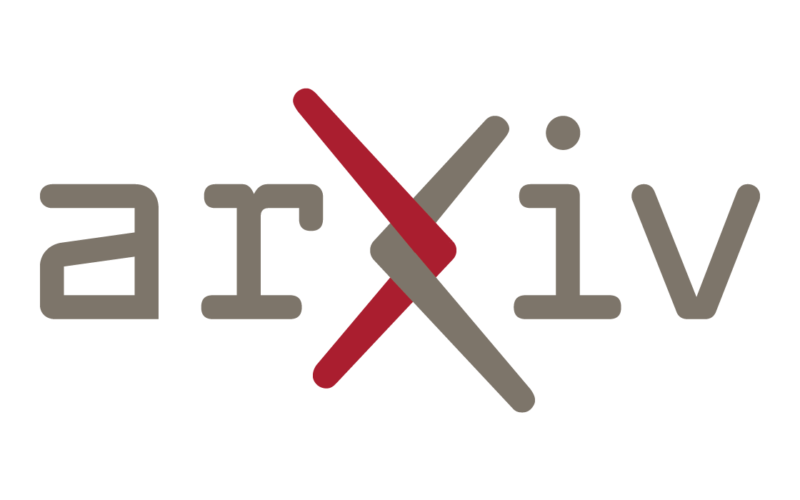