arXiv:2406.09454v1 Announce Type: new
Abstract: Multi-modal learning has significantly advanced generative AI, especially in vision-language modeling. Innovations like GPT-4V and open-source projects such as LLaVA have enabled robust conversational agents capable of zero-shot task completions. However, applying these technologies in the biomedical field presents unique challenges. Recent initiatives like LLaVA-Med have started to adapt instruction-tuning for biomedical contexts using large datasets such as PMC-15M. Our research offers three key contributions: (i) we present a new instruct dataset enriched with medical image-text pairs from Claude3-Opus and LLaMA3 70B, (ii) we propose a novel image encoding strategy using hierarchical representations to improve fine-grained biomedical visual comprehension, and (iii) we develop the Llama3-Med model, which achieves state-of-the-art zero-shot performance on biomedical visual question answering benchmarks, with an average performance improvement of over 10% compared to previous methods. These advancements provide more accurate and reliable tools for medical professionals, bridging gaps in current multi-modal conversational assistants and promoting further innovations in medical AI.
Source link
lol
Advancing High Resolution Vision-Language Models in Biomedicine
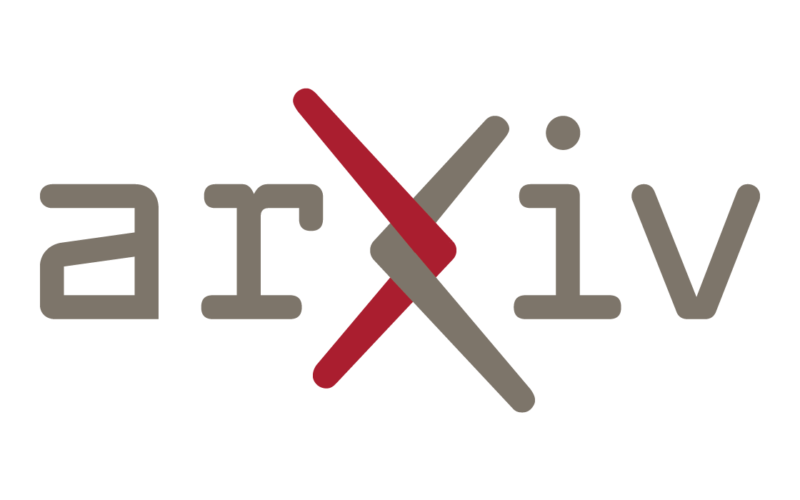