arXiv:2501.02021v1 Announce Type: new
Abstract: Graph classification plays a pivotal role in various domains, including pathology, where images can be represented as graphs.In this domain, images can be represented as graphs, where nodes might represent individual nuclei, and edges capture the spatial or functional relationships between them. Often, the overall label of the graph, such as a cancer type or disease state, is determined by patterns within smaller, localized regions of the image. This work introduces a weakly-supervised graph classification framework leveraging two subgraph extraction techniques: (1) Sliding-window approach (2) BFS-based approach. Subgraphs are processed using a Graph Attention Network (GAT), which employs attention mechanisms to identify the most informative subgraphs for classification. Weak supervision is achieved by propagating graph-level labels to subgraphs, eliminating the need for detailed subgraph annotations.
Source link
lol
Weakly Supervised Learning on Large Graphs
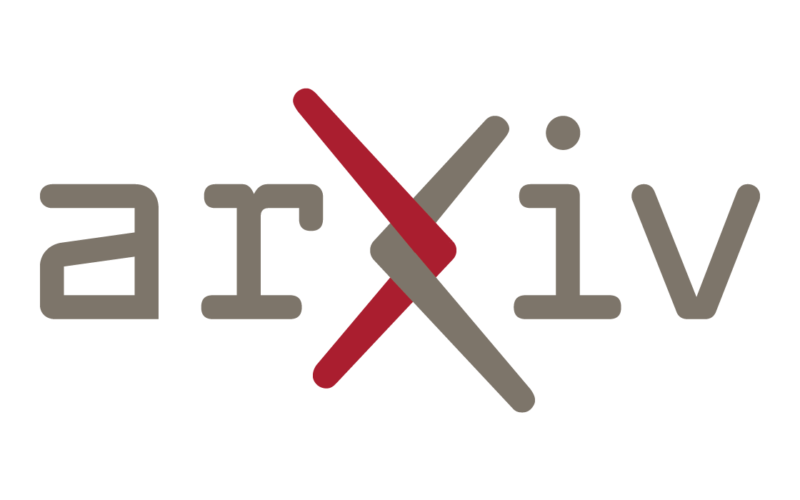