arXiv:2501.01986v1 Announce Type: new
Abstract: The increasing demand to process long and high-resolution videos significantly burdens Large Vision-Language Models (LVLMs) due to the enormous number of visual tokens. Existing token reduction methods primarily focus on importance-based token pruning, which overlooks the redundancy caused by frame resemblance and repetitive visual elements. In this paper, we analyze the high vision token similarities in LVLMs. We reveal that token similarity distribution condenses as layers deepen while maintaining ranking consistency. Leveraging the unique properties of similarity over importance, we introduce FrameFusion, a novel approach that combines similarity-based merging with importance-based pruning for better token reduction in LVLMs. FrameFusion identifies and merges similar tokens before pruning, opening up a new perspective for token reduction. We evaluate FrameFusion on diverse LVLMs, including Llava-Video-{7B,32B,72B}, and MiniCPM-V-8B, on video understanding, question-answering, and retrieval benchmarks. Experiments show that FrameFusion reduces vision tokens by 70$%$, achieving 3.4-4.4x LLM speedups and 1.6-1.9x end-to-end speedups, with an average performance impact of less than 3$%$. Our code is available at https://github.com/thu-nics/FrameFusion.
Source link
lol
FrameFusion: Combining Similarity and Importance for Video Token Reduction on Large Visual Language Models
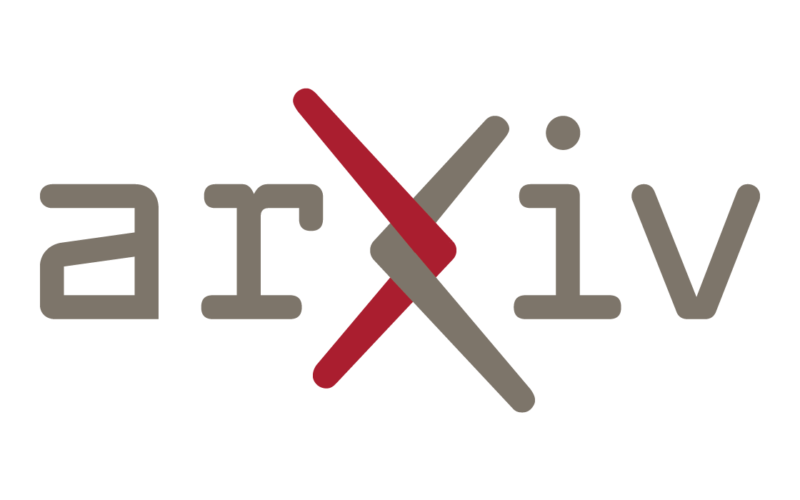