arXiv:2501.00124v1 Announce Type: new
Abstract: Diffusionmodels(DMs)havedemonstratedremarkableachievements in synthesizing images of high fidelity and diversity. However, the extensive computational requirements and slow generative speed of diffusion models have limited their widespread adoption. In this paper, we propose a novel post-training quantization for diffusion models (PQD), which is a time-aware optimization framework for diffusion models based on post-training quantization. The proposed framework optimizes the inference process by selecting representative samples and conducting time-aware calibration. Experimental results show that our proposed method is able to directly quantize full-precision diffusion models into 8-bit or 4-bit models while maintaining comparable performance in a training-free manner, achieving a few FID change on ImageNet for unconditional image generation. Our approach demonstrates compatibility and can also be applied to 512×512 text-guided image generation for the first time.
Source link
lol
PQD: Post-training Quantization for Efficient Diffusion Models
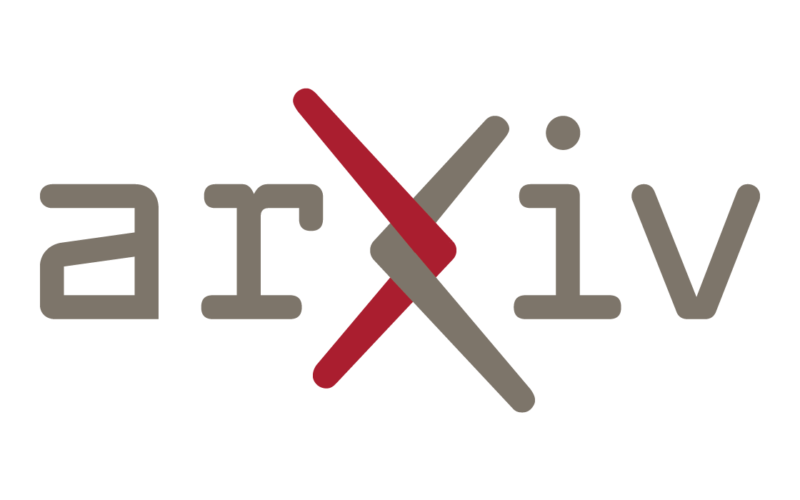