arXiv:2501.00052v1 Announce Type: new
Abstract: Mean Field Control Games (MFCGs) provide a powerful theoretical framework for analyzing systems of infinitely many interacting agents, blending elements from Mean Field Games (MFGs) and Mean Field Control (MFC). However, solving the coupled Hamilton-Jacobi-Bellman and Fokker-Planck equations that characterize MFCG equilibria remains a significant computational challenge, particularly in high-dimensional or complex environments.
This paper presents a scalable deep Reinforcement Learning (RL) approach to approximate equilibrium solutions of MFCGs. Building on previous works, We reformulate the infinite-agent stochastic control problem as a Markov Decision Process, where each representative agent interacts with the evolving mean field distribution. We use the actor-critic based algorithm from a previous paper (Angiuli et.al., 2024) as the baseline and propose several versions of more scalable and efficient algorithms, utilizing techniques including parallel sample collection (batching); mini-batching; target network; proximal policy optimization (PPO); generalized advantage estimation (GAE); and entropy regularization. By leveraging these techniques, we effectively improved the efficiency, scalability, and training stability of the baseline algorithm.
We evaluate our method on a linear-quadratic benchmark problem, where an analytical solution to the MFCG equilibrium is available. Our results show that some versions of our proposed approach achieve faster convergence and closely approximate the theoretical optimum, outperforming the baseline algorithm by an order of magnitude in sample efficiency. Our work lays the foundation for adapting deep RL to solve more complicated MFCGs closely related to real life, such as large-scale autonomous transportation systems, multi-firm economic competition, and inter-bank borrowing problems.
Source link
lol
Efficient and Scalable Deep Reinforcement Learning for Mean Field Control Games
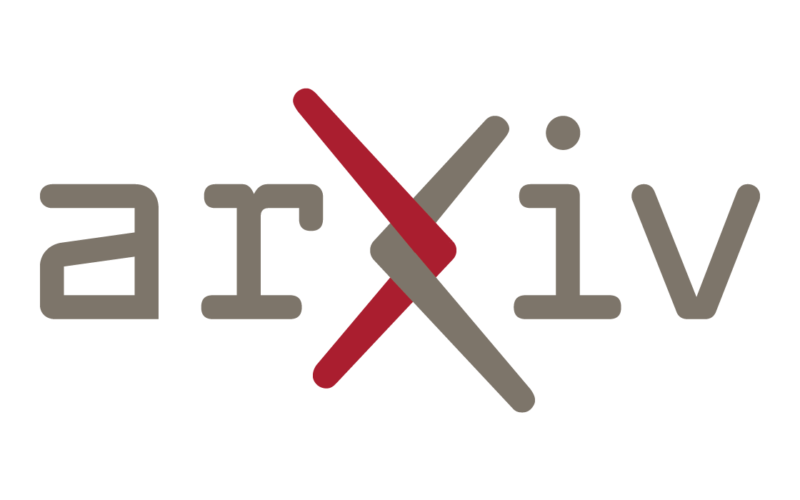