arXiv:2501.00243v1 Announce Type: new
Abstract: Ultra-fine-grained image recognition (UFGIR) is a challenging task that involves classifying images within a macro-category. While traditional FGIR deals with classifying different species, UFGIR goes beyond by classifying sub-categories within a species such as cultivars of a plant. In recent times the usage of Vision Transformer-based backbones has allowed methods to obtain outstanding recognition performances in this task but this comes at a significant cost in terms of computation specially since this task significantly benefits from incorporating higher resolution images. Therefore, techniques such as token reduction have emerged to reduce the computational cost. However, dropping tokens leads to loss of essential information for fine-grained categories, specially as the token keep rate is reduced. Therefore, to counteract the loss of information brought by the usage of token reduction we propose a novel Cross-Layer Aggregation Classification Head and a Cross-Layer Cache mechanism to recover and access information from previous layers in later locations. Extensive experiments covering more than 2000 runs across diverse settings including 5 datasets, 9 backbones, 7 token reduction methods, 5 keep rates, and 2 image sizes demonstrate the effectiveness of the proposed plug-and-play modules and allow us to push the boundaries of accuracy vs cost for UFGIR by reducing the kept tokens to extremely low ratios of up to 10% while maintaining a competitive accuracy to state-of-the-art models. Code is available at: url{https://github.com/arkel23/CLCA}
Source link
lol
Cross-Layer Cache Aggregation for Token Reduction in Ultra-Fine-Grained Image Recognition
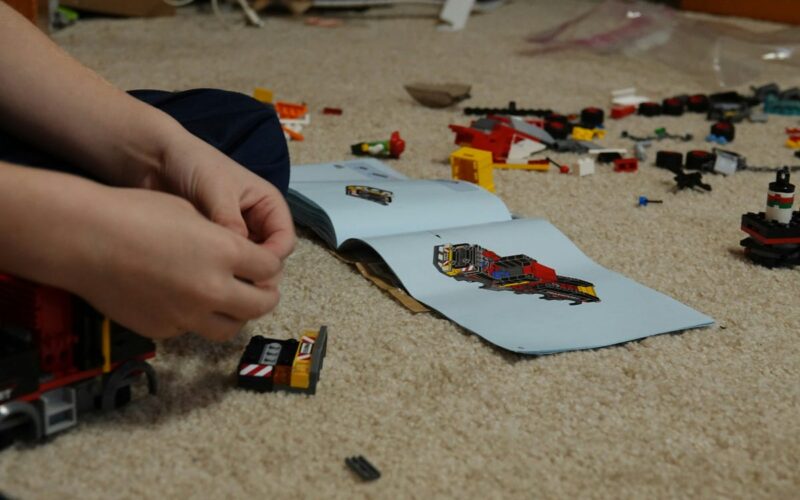