arXiv:2501.00437v1 Announce Type: cross
Abstract: Recently, zero-shot image captioning has gained increasing attention, where only text data is available for training. The remarkable progress in text-to-image diffusion model presents the potential to resolve this task by employing synthetic image-caption pairs generated by this pre-trained prior. Nonetheless, the defective details in the salient regions of the synthetic images introduce semantic misalignment between the synthetic image and text, leading to compromised results. To address this challenge, we propose a novel Patch-wise Cross-modal feature Mix-up (PCM) mechanism to adaptively mitigate the unfaithful contents in a fine-grained manner during training, which can be integrated into most of encoder-decoder frameworks, introducing our PCM-Net. Specifically, for each input image, salient visual concepts in the image are first detected considering the image-text similarity in CLIP space. Next, the patch-wise visual features of the input image are selectively fused with the textual features of the salient visual concepts, leading to a mixed-up feature map with less defective content. Finally, a visual-semantic encoder is exploited to refine the derived feature map, which is further incorporated into the sentence decoder for caption generation. Additionally, to facilitate the model training with synthetic data, a novel CLIP-weighted cross-entropy loss is devised to prioritize the high-quality image-text pairs over the low-quality counterparts. Extensive experiments on MSCOCO and Flickr30k datasets demonstrate the superiority of our PCM-Net compared with state-of-the-art VLMs-based approaches. It is noteworthy that our PCM-Net ranks first in both in-domain and cross-domain zero-shot image captioning. The synthetic dataset SynthImgCap and code are available at https://jianjieluo.github.io/SynthImgCap.
Source link
lol
Unleashing Text-to-Image Diffusion Prior for Zero-Shot Image Captioning
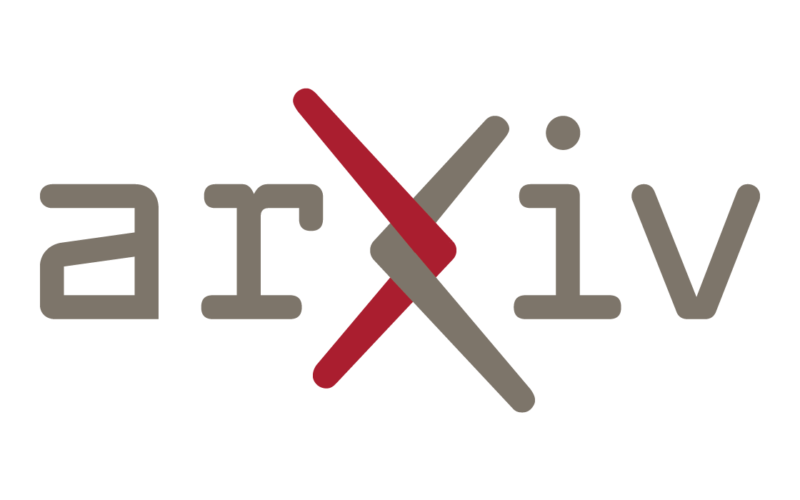