arXiv:2412.20043v1 Announce Type: new
Abstract: Large language models (LLMs) demonstrate the ability to learn in-context, offering a potential solution for scientific information extraction, which often contends with challenges such as insufficient training data and the high cost of annotation processes. Given that the selection of in-context examples can significantly impact performance, it is crucial to design a proper method to sample the efficient ones. In this paper, we propose STAYKATE, a static-dynamic hybrid selection method that combines the principles of representativeness sampling from active learning with the prevalent retrieval-based approach. The results across three domain-specific datasets indicate that STAYKATE outperforms both the traditional supervised methods and existing selection methods. The enhancement in performance is particularly pronounced for entity types that other methods pose challenges.
Source link
lol
STAYKATE: Hybrid In-Context Example Selection Combining Representativeness Sampling and Retrieval-based Approach — A Case Study on Science Domains
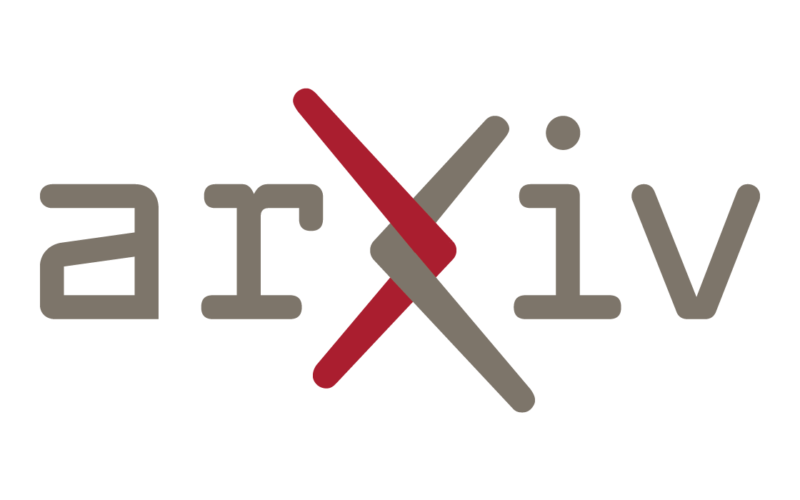