arXiv:2412.18820v1 Announce Type: new
Abstract: Trajectory anomaly detection, aiming to estimate the anomaly risk of trajectories given the Source-Destination (SD) pairs, has become a critical problem for many real-world applications. Existing solutions directly train a generative model for observed trajectories and calculate the conditional generative probability $P({T}|{C})$ as the anomaly risk, where ${T}$ and ${C}$ represent the trajectory and SD pair respectively. However, we argue that the observed trajectories are confounded by road network preference which is a common cause of both SD distribution and trajectories. Existing methods ignore this issue limiting their generalization ability on out-of-distribution trajectories. In this paper, we define the debiased trajectory anomaly detection problem and propose a causal implicit generative model, namely CausalTAD, to solve it. CausalTAD adopts do-calculus to eliminate the confounding bias of road network preference and estimates $P({T}|do({C}))$ as the anomaly criterion. Extensive experiments show that CausalTAD can not only achieve superior performance on trained trajectories but also generally improve the performance of out-of-distribution data, with improvements of $2.1% sim 5.7%$ and $10.6% sim 32.7%$ respectively.
Source link
lol
CausalTAD: Causal Implicit Generative Model for Debiased Online Trajectory Anomaly Detection
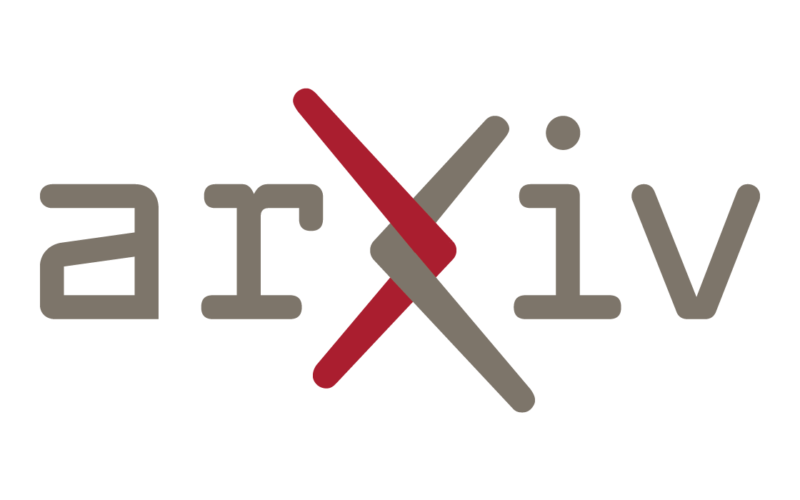