arXiv:2412.18621v1 Announce Type: new
Abstract: Pre-trained Large Language Models (LLMs) have demonstrated remarkable capabilities but also pose risks by learning and generating copyrighted material, leading to significant legal and ethical concerns. In a potential real-world scenario, model owners may need to continuously address copyright infringement in order to address requests for content removal that emerge at different time points. One potential way of addressing this is via sequential unlearning, where copyrighted content is removed sequentially as new requests arise. Despite its practical relevance, sequential unlearning in the context of copyright infringement has not been rigorously explored in existing literature. To address this gap, we propose Stable Sequential Unlearning (SSU), a novel framework designed to unlearn copyrighted content from LLMs over multiple time steps. Our approach works by identifying and removing specific weight updates in the model’s parameters that correspond to copyrighted content using task vectors. We improve unlearning efficacy by introducing random labeling loss and ensuring the model retains its general-purpose knowledge by adjusting targeted parameters with gradient-based weight saliency. Extensive experimental results show that SSU sometimes achieves an effective trade-off between unlearning efficacy and general-purpose language abilities, outperforming existing baselines, but it’s not a cure-all for unlearning copyrighted material.
Source link
lol
Investigating the Feasibility of Mitigating Potential Copyright Infringement via Large Language Model Unlearning
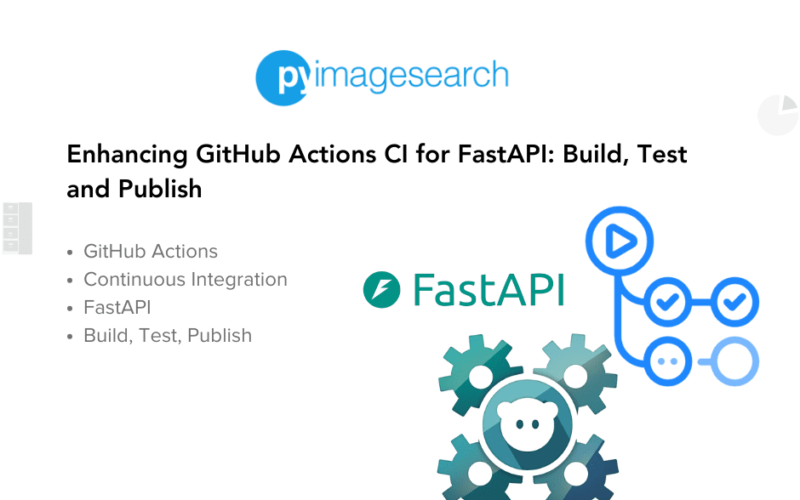