arXiv:2412.18786v1 Announce Type: new
Abstract: Understanding thermal stress evolution in metal additive manufacturing (AM) is crucial for producing high-quality components. Recent advancements in machine learning (ML) have shown great potential for modeling complex multiphysics problems in metal AM. While physics-based simulations face the challenge of high computational costs, conventional data-driven ML models require large, labeled training datasets to achieve accurate predictions. Unfortunately, generating large datasets for ML model training through time-consuming experiments or high-fidelity simulations is highly expensive in metal AM. To address these challenges, this study introduces a physics-informed neural network (PINN) framework that incorporates governing physical laws into deep neural networks (NNs) to predict temperature and thermal stress evolution during the laser metal deposition (LMD) process. The study also discusses the enhanced accuracy and efficiency of the PINN model when supplemented with small simulation data. Furthermore, it highlights the PINN transferability, enabling fast predictions with a set of new process parameters using a pre-trained PINN model as an online soft sensor, significantly reducing computation time compared to physics-based numerical models while maintaining accuracy.
Source link
lol
Thermal-Mechanical Physics Informed Deep Learning For Fast Prediction of Thermal Stress Evolution in Laser Metal Deposition
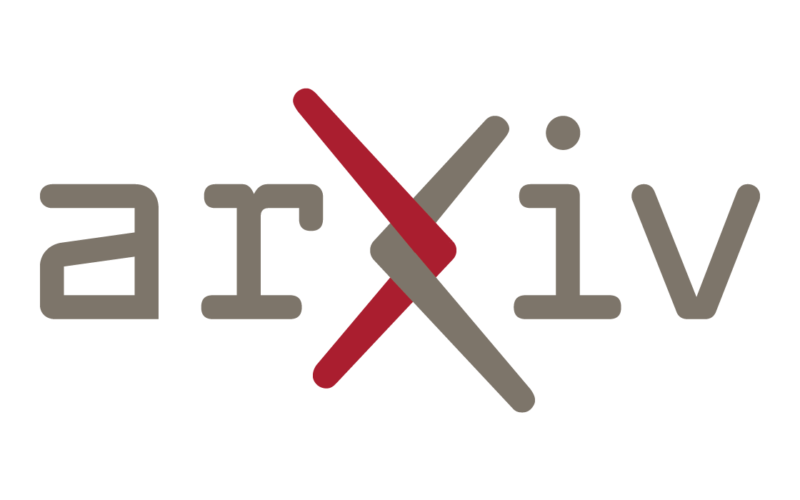