arXiv:2412.17982v1 Announce Type: new
Abstract: Spatially varying regularization accommodates the deformation variations that may be necessary for different anatomical regions during deformable image registration. Historically, optimization-based registration models have harnessed spatially varying regularization to address anatomical subtleties. However, most modern deep learning-based models tend to gravitate towards spatially invariant regularization, wherein a homogenous regularization strength is applied across the entire image, potentially disregarding localized variations. In this paper, we propose a hierarchical probabilistic model that integrates a prior distribution on the deformation regularization strength, enabling the end-to-end learning of a spatially varying deformation regularizer directly from the data. The proposed method is straightforward to implement and easily integrates with various registration network architectures. Additionally, automatic tuning of hyperparameters is achieved through Bayesian optimization, allowing efficient identification of optimal hyperparameters for any given registration task. Comprehensive evaluations on publicly available datasets demonstrate that the proposed method significantly improves registration performance and enhances the interpretability of deep learning-based registration, all while maintaining smooth deformations.
Source link
lol
Unsupervised learning of spatially varying regularization for diffeomorphic image registration
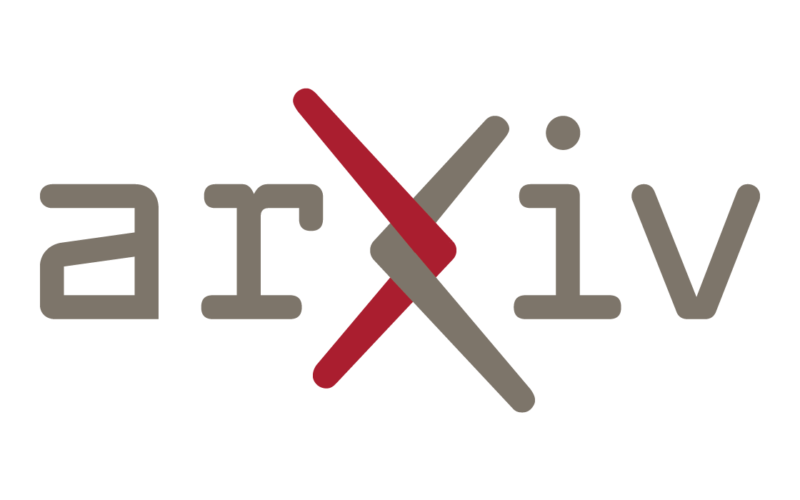