arXiv:2412.16213v1 Announce Type: new
Abstract: The increasing deployment of AI models in critical applications has exposed them to significant risks from adversarial attacks. While adversarial vulnerabilities in 2D vision models have been extensively studied, the threat landscape for 3D generative models, such as Neural Radiance Fields (NeRF), remains underexplored. This work introduces textit{AdvIRL}, a novel framework for crafting adversarial NeRF models using Instant Neural Graphics Primitives (Instant-NGP) and Reinforcement Learning. Unlike prior methods, textit{AdvIRL} generates adversarial noise that remains robust under diverse 3D transformations, including rotations and scaling, enabling effective black-box attacks in real-world scenarios. Our approach is validated across a wide range of scenes, from small objects (e.g., bananas) to large environments (e.g., lighthouses). Notably, targeted attacks achieved high-confidence misclassifications, such as labeling a banana as a slug and a truck as a cannon, demonstrating the practical risks posed by adversarial NeRFs. Beyond attacking, textit{AdvIRL}-generated adversarial models can serve as adversarial training data to enhance the robustness of vision systems. The implementation of textit{AdvIRL} is publicly available at url{https://github.com/Tommy-Nguyen-cpu/AdvIRL/tree/MultiView-Clean}, ensuring reproducibility and facilitating future research.
Source link
lol
AdvIRL: Reinforcement Learning-Based Adversarial Attacks on 3D NeRF Models
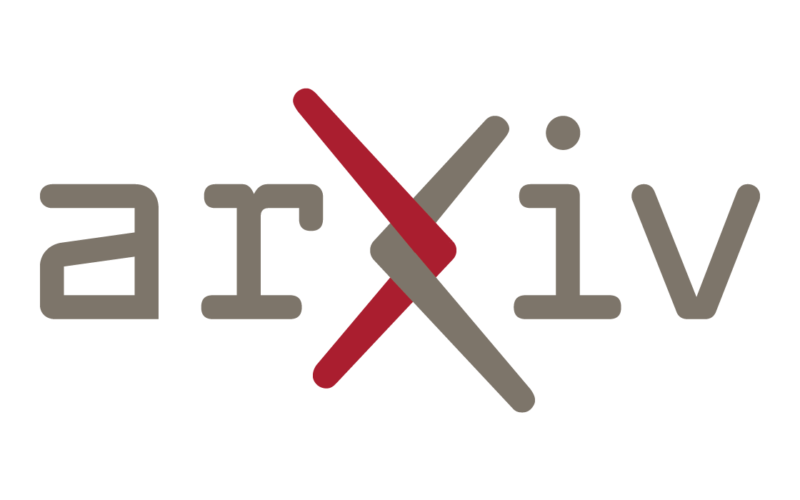