arXiv:2412.15322v1 Announce Type: new
Abstract: We propose to synthesize high-quality and synchronized audio, given video and optional text conditions, using a novel multimodal joint training framework MMAudio. In contrast to single-modality training conditioned on (limited) video data only, MMAudio is jointly trained with larger-scale, readily available text-audio data to learn to generate semantically aligned high-quality audio samples. Additionally, we improve audio-visual synchrony with a conditional synchronization module that aligns video conditions with audio latents at the frame level. Trained with a flow matching objective, MMAudio achieves new video-to-audio state-of-the-art among public models in terms of audio quality, semantic alignment, and audio-visual synchronization, while having a low inference time (1.23s to generate an 8s clip) and just 157M parameters. MMAudio also achieves surprisingly competitive performance in text-to-audio generation, showing that joint training does not hinder single-modality performance. Code and demo are available at: https://hkchengrex.github.io/MMAudio
Source link
lol
Taming Multimodal Joint Training for High-Quality Video-to-Audio Synthesis
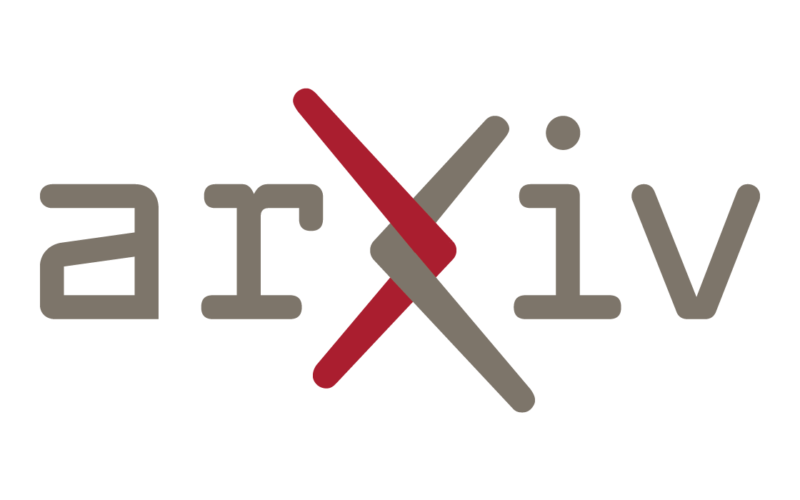