arXiv:2412.14226v1 Announce Type: new
Abstract: Federated learning (FL) is a machine learning methodology that involves the collaborative training of a global model across multiple decentralized clients in a privacy-preserving way. Several FL methods are introduced to tackle communication inefficiencies but do not address how to sample participating clients in each round effectively and in a privacy-preserving manner. In this paper, we propose textit{FedSTaS}, a client and data-level sampling method inspired by textit{FedSTS} and textit{FedSampling}. In each federated learning round, textit{FedSTaS} stratifies clients based on their compressed gradients, re-allocate the number of clients to sample using an optimal Neyman allocation, and sample local data from each participating clients using a data uniform sampling strategy. Experiments on three datasets show that textit{FedSTaS} can achieve higher accuracy scores than those of textit{FedSTS} within a fixed number of training rounds.
Source link
lol
FedSTaS: Client Stratification and Client Level Sampling for Efficient Federated Learning
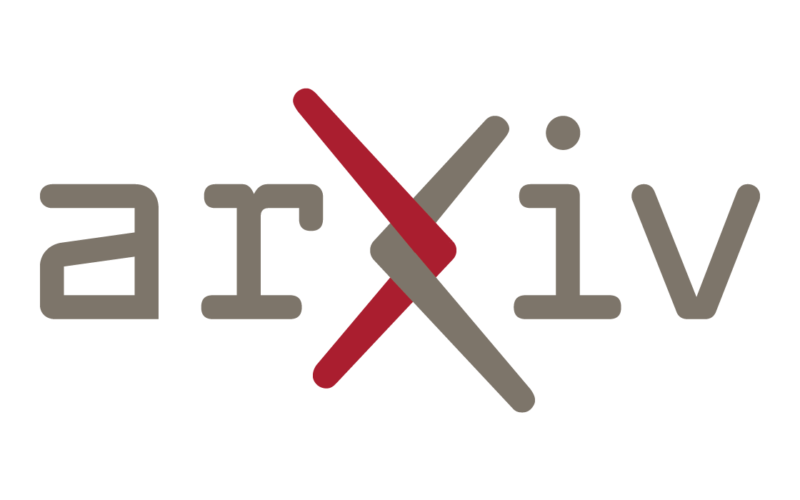