arXiv:2412.13232v1 Announce Type: new
Abstract: Due to the superior ability of global dependency, transformer and its variants have become the primary choice in Masked Time-series Modeling (MTM) towards time-series classification task. In this paper, we experimentally analyze that existing transformer-based MTM methods encounter with two under-explored issues when dealing with time series data: (1) they encode features by performing long-dependency ensemble averaging, which easily results in rank collapse and feature homogenization as the layer goes deeper; (2) they exhibit distinct priorities in fitting different frequency components contained in the time-series, inevitably leading to spectrum energy imbalance of encoded feature. To tackle these issues, we propose an auxiliary content-aware balanced decoder (CBD) to optimize the encoding quality in the spectrum space within masked modeling scheme. Specifically, the CBD iterates on a series of fundamental blocks, and thanks to two tailored units, each block could progressively refine the masked representation via adjusting the interaction pattern based on local content variations of time-series and learning to recalibrate the energy distribution across different frequency components. Moreover, a dual-constraint loss is devised to enhance the mutual optimization of vanilla decoder and our CBD. Extensive experimental results on ten time-series classification datasets show that our method nearly surpasses a bunch of baselines. Meanwhile, a series of explanatory results are showcased to sufficiently demystify the behaviors of our method.
Source link
lol
Content-aware Balanced Spectrum Encoding in Masked Modeling for Time Series Classification
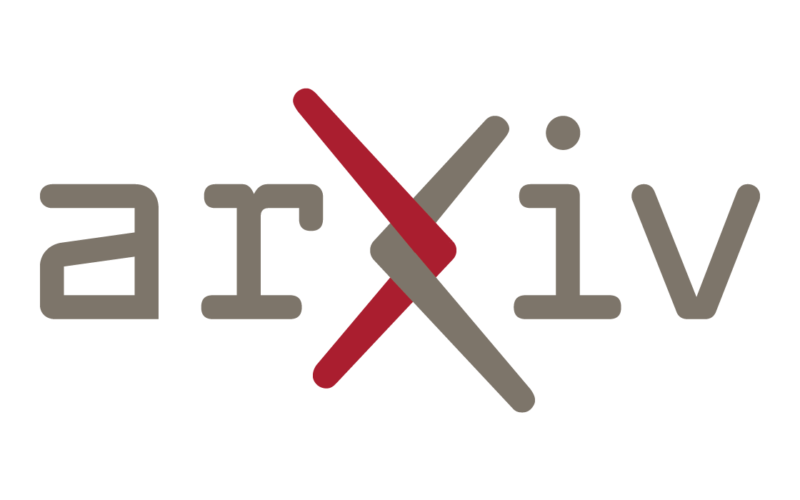