arXiv:2412.13236v1 Announce Type: new
Abstract: Early exiting is an effective paradigm for improving the inference efficiency of pre-trained language models (PLMs) by dynamically adjusting the number of executed layers for each sample. However, in most existing works, easy and hard samples are treated equally by each classifier during training, which neglects the test-time early exiting behavior, leading to inconsistency between training and testing. Although some methods have tackled this issue under a fixed speed-up ratio, the challenge of flexibly adjusting the speed-up ratio while maintaining consistency between training and testing is still under-explored. To bridge the gap, we propose a novel Consistency-Oriented Signal-based Early Exiting (COSEE) framework, which leverages a calibrated sample weighting mechanism to enable each classifier to emphasize the samples that are more likely to exit at that classifier under various acceleration scenarios. Extensive experiments on the GLUE benchmark demonstrate the effectiveness of our COSEE across multiple exiting signals and backbones, yielding a better trade-off between performance and efficiency.
Source link
lol
COSEE: Consistency-Oriented Signal-Based Early Exiting via Calibrated Sample Weighting Mechanism
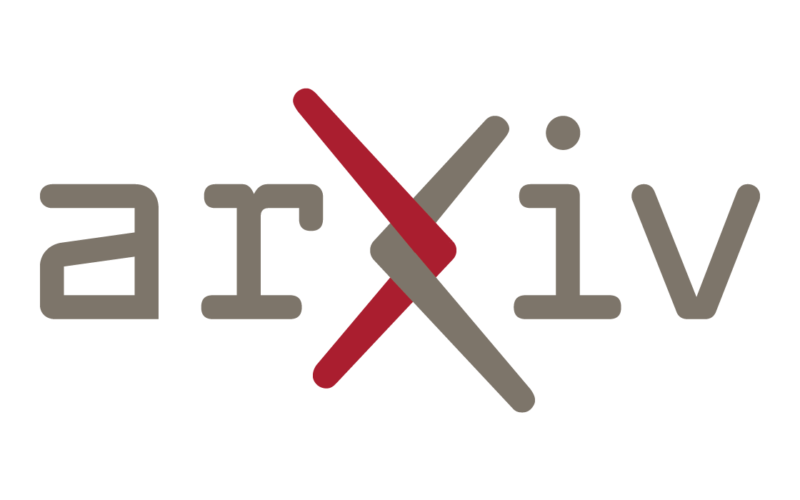