arXiv:2412.12160v1 Announce Type: new
Abstract: Wind power forecasting plays a critical role in modern energy systems, facilitating the integration of renewable energy sources into the power grid. Accurate prediction of wind energy output is essential for managing the inherent intermittency of wind power, optimizing energy dispatch, and ensuring grid stability. This paper proposes the use of Deep Neural Network (DNN)-based predictive models that leverage climate datasets, including wind speed, atmospheric pressure, temperature, and other meteorological variables, to improve the accuracy of wind power simulations. In particular, we focus on the Coupled Model Intercomparison Project (CMIP) datasets, which provide climate projections, as inputs for training the DNN models. These models aim to capture the complex nonlinear relationships between the CMIP-based climate data and actual wind power generation at wind farms located in Germany. Our study compares various DNN architectures, specifically Multilayer Perceptron (MLP), Long Short-Term Memory (LSTM) networks, and Transformer-enhanced LSTM models, to identify the best configuration among these architectures for climate-aware wind power simulation. The implementation of this framework involves the development of a Python package (CADNN) designed to support multiple tasks, including statistical analysis of the climate data, data visualization, preprocessing, DNN training, and performance evaluation. We demonstrate that the DNN models, when integrated with climate data, significantly enhance forecasting accuracy. This climate-aware approach offers a deeper understanding of the time-dependent climate patterns that influence wind power generation, providing more accurate predictions and making it adaptable to other geographical regions.
Source link
lol
Climate Aware Deep Neural Networks (CADNN) for Wind Power Simulation
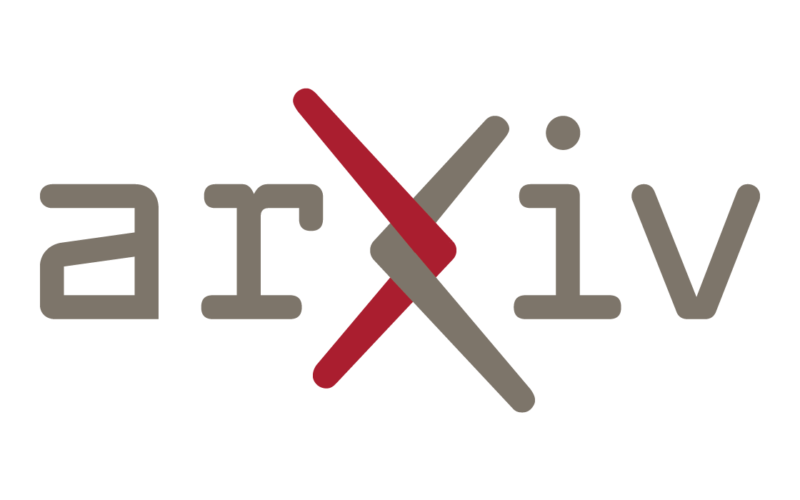