arXiv:2406.08636v1 Announce Type: new
Abstract: Machine learning (ML) models can make decisions based on large amounts of data, but they can be missing personal knowledge available to human users about whom predictions are made. For example, a model trained to predict psychiatric outcomes may know nothing about a patient’s social support system, and social support may look different for different patients. In this work, we introduce the problem of human feature integration, which provides a way to incorporate important personal-knowledge from users without domain expertise into ML predictions. We characterize this problem through illustrative user stories and comparisons to existing approaches; we formally describe this problem in a way that paves the ground for future technical solutions; and we provide a proof-of-concept study of a simple version of a solution to this problem in a semi-realistic setting.
Source link
lol
Towards Integrating Personal Knowledge into Test-Time Predictions
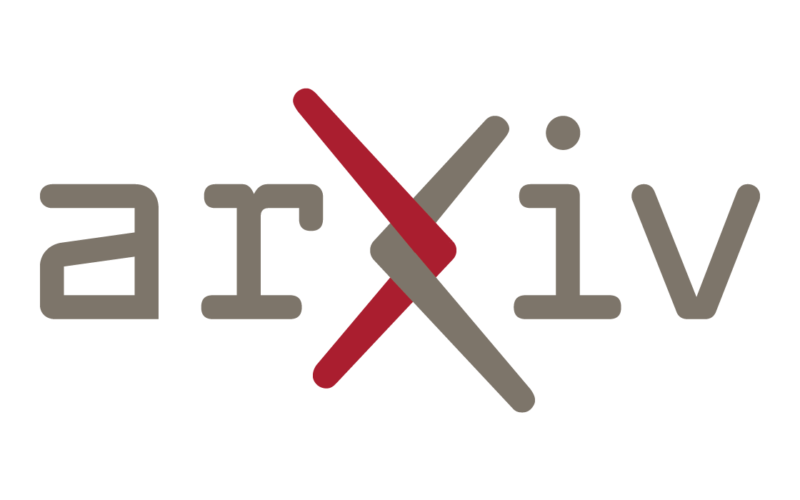