arXiv:2412.12151v1 Announce Type: new
Abstract: The tool-use ability of Large Language Models (LLMs) has a profound impact on a wide range of industrial applications. However, LLMs’ self-control and calibration capability in appropriately using tools remains understudied. The problem is consequential as it raises potential risks of degraded performance and poses a threat to the trustworthiness of the models. In this paper, we conduct a study on a family of state-of-the-art LLMs on three datasets with two mainstream tool-use frameworks. Our study reveals the tool-abuse behavior of LLMs, a tendency for models to misuse tools with overconfidence. We also find that this is a common issue regardless of model capability. Accordingly, we propose a novel approach, textit{SMARTCAL}, to mitigate the observed issues, and our results show an average of 8.6 percent increase in the QA performance and a 21.6 percent decrease in Expected Calibration Error (ECE) compared to baseline models.
Source link
lol
SMARTCAL: An Approach to Self-Aware Tool-Use Evaluation and Calibration
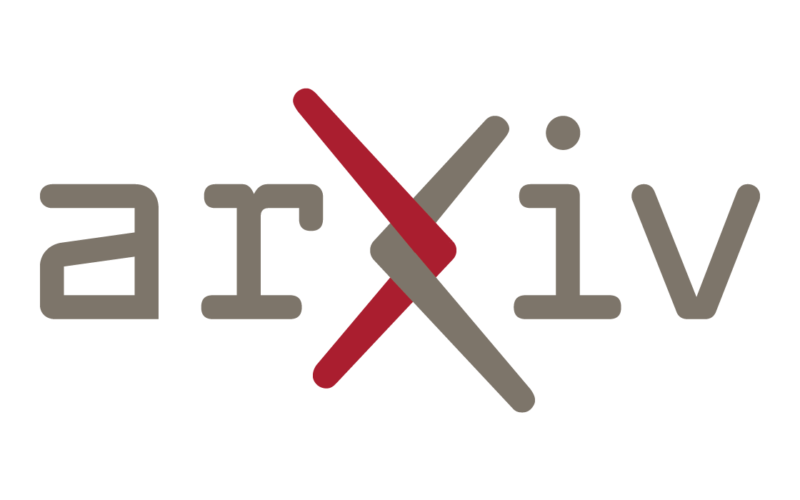