arXiv:2412.11303v1 Announce Type: cross
Abstract: We study the problem of drawing samples from a logconcave distribution truncated on a polytope, motivated by computational challenges in Bayesian statistical models with indicator variables, such as probit regression. Building on interior point methods and the Dikin walk for sampling from uniform distributions, we analyze the mixing time of regularized Dikin walks. Our contributions are threefold. First, for a logconcave and log-smooth distribution with condition number $kappa$, truncated on a polytope in $mathbb{R}^n$ defined with $m$ linear constraints, we prove that the soft-threshold Dikin walk mixes in $widetilde{O}((m+kappa)n)$ iterations from a warm initialization. It improves upon prior work which required the polytope to be bounded and involved a bound dependent on the radius of the bounded region. Moreover, we introduce the regularized Dikin walk using Lewis weights for approximating the John ellipsoid. We show that it mixes in $widetilde{O}((n^{2.5}+kappa n)$. Second, we extend the mixing time guarantees mentioned above to weakly log-concave distributions truncated on polytopes, provided that they have a finite covariance matrix. Third, going beyond worst-case mixing time analysis, we demonstrate that soft-threshold Dikin walk can mix significantly faster when only a limited number of constraints intersect the high-probability mass of the distribution, improving the $widetilde{O}((m+kappa)n)$ upper bound to $widetilde{O}(m + kappa n)$. Additionally, per-iteration complexity of regularized Dikin walk and ways to generate a warm initialization are discussed to facilitate practical implementation.
Source link
lol
Regularized Dikin Walks for Sampling Truncated Logconcave Measures, Mixed Isoperimetry and Beyond Worst-Case Analysis
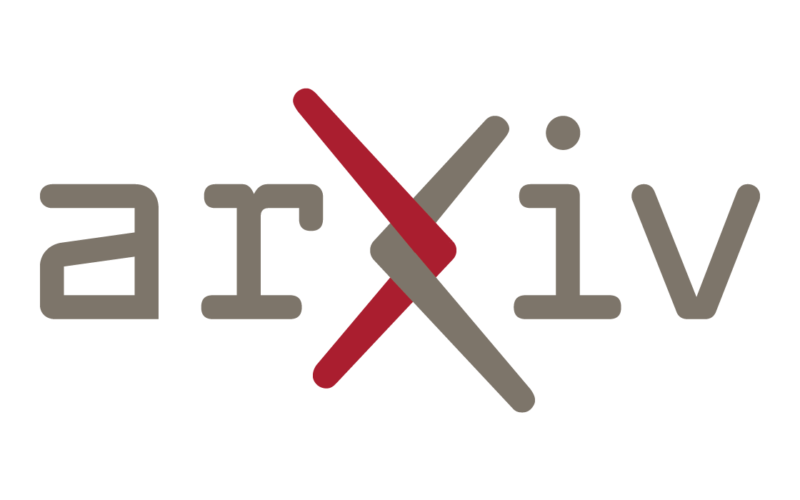