arXiv:2406.07716v1 Announce Type: new
Abstract: The purpose of the Insect Detection System for Crop and Plant Health is to keep an eye out for and identify insect infestations in farming areas. By utilizing cutting-edge technology like computer vision and machine learning, the system seeks to identify hazardous insects early and accurately. This would enable prompt response to save crops and maintain optimal plant health. The Method of this study includes Data Acquisition, Preprocessing, Data splitting, Model Implementation and Model evaluation. Different models like MobileNetV2, ResNet152V2, Xecption, Custom CNN was used in this study. In order to categorize insect photos, a Convolutional Neural Network (CNN) based on the ResNet152V2 architecture is constructed and evaluated in this work. Achieving 99% training accuracy and 97% testing accuracy, ResNet152V2 demonstrates superior performance among four implemented models. The results highlight its potential for real-world applications in insect classification and entomology studies, emphasizing efficiency and accuracy. To ensure food security and sustain agricultural output globally, finding insects is crucial. Cutting-edge technology, such as ResNet152V2 models, greatly influence automating and improving the accuracy of insect identification. Efficient insect detection not only minimizes crop losses but also enhances agricultural productivity, contributing to sustainable food production. This underscores the pivotal role of technology in addressing challenges related to global food security.
Source link
lol
Unleashing the Power of Transfer Learning Model for Sophisticated Insect Detection: Revolutionizing Insect Classification
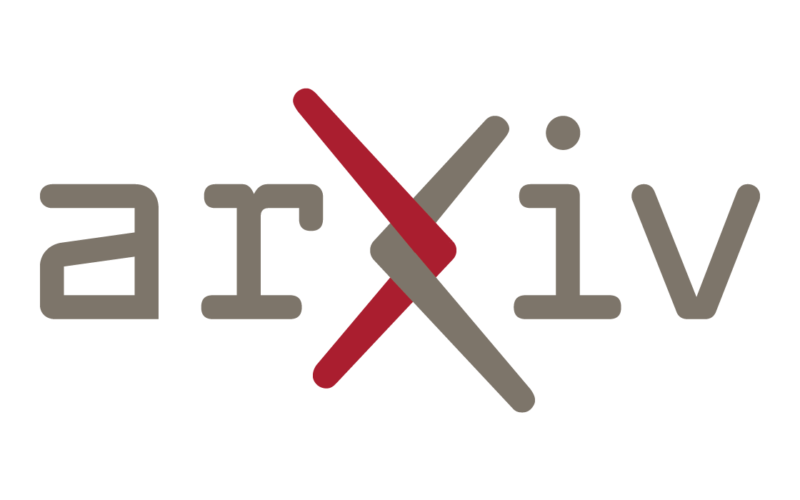