arXiv:2412.08127v1 Announce Type: new
Abstract: It has been widely observed that language models (LMs) respond in predictable ways to algorithmically generated prompts that are seemingly unintelligible. This is both a sign that we lack a full understanding of how LMs work, and a practical challenge, because opaqueness can be exploited for harmful uses of LMs, such as jailbreaking. We present the first thorough analysis of opaque machine-generated prompts, or autoprompts, pertaining to 3 LMs of different sizes and families. We find that machine-generated prompts are characterized by a last token that is often intelligible and strongly affects the generation. A small but consistent proportion of the previous tokens are fillers that probably appear in the prompt as a by-product of the fact that the optimization process fixes the number of tokens. The remaining tokens tend to have at least a loose semantic relation with the generation, although they do not engage in well-formed syntactic relations with it. We find moreover that some of the ablations we applied to machine-generated prompts can also be applied to natural language sequences, leading to similar behavior, suggesting that autoprompts are a direct consequence of the way in which LMs process linguistic inputs in general.
Source link
lol
Evil twins are not that evil: Qualitative insights into machine-generated prompts
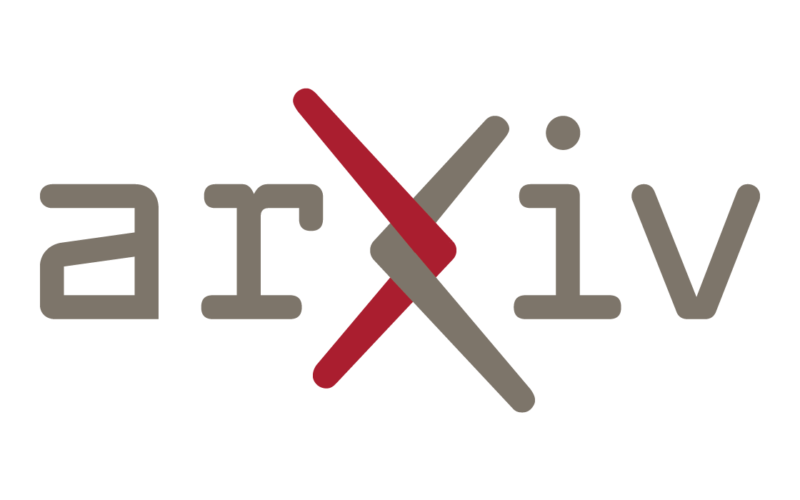