arXiv:2412.06840v1 Announce Type: new
Abstract: The fast fashion industry suffers from significant environmental impacts due to overproduction and unsold inventory. Accurately predicting sales volumes for unreleased products could significantly improve efficiency and resource utilization. However, predicting performance for entirely new items is challenging due to the lack of historical data and rapidly changing trends, and existing deterministic models often struggle with domain shifts when encountering items outside the training data distribution. The recently proposed diffusion models address this issue using a continuous-time diffusion process. This allows us to simulate how new items are adopted, reducing the impact of domain shift challenges faced by deterministic models. As a result, in this paper, we propose MDiFF: a novel two-step multimodal diffusion models-based pipeline for New Fashion Product Performance Forecasting (NFPPF). First, we use a score-based diffusion model to predict multiple future sales for different clothes over time. Then, we refine these multiple predictions with a lightweight Multi-layer Perceptron (MLP) to get the final forecast. MDiFF leverages the strengths of both architectures, resulting in the most accurate and efficient forecasting system for the fast-fashion industry at the state-of-the-art. The code can be found at https://github.com/intelligolabs/MDiFF.
Source link
lol
MDiFF: Exploiting Multimodal Score-based Diffusion Models for New Fashion Product Performance Forecasting
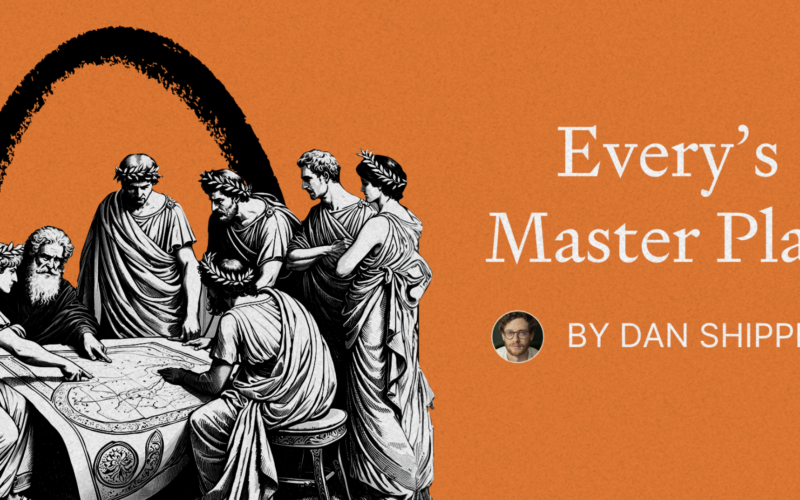