arXiv:2412.03814v1 Announce Type: new
Abstract: Image Restoration aims to restore degraded images, with deep learning, especially CNNs and Transformers, enhancing performance. However, there’s a lack of a unified training benchmark for IR. We identified a bias in image complexity between training and testing datasets, affecting restoration quality. To address this, we created ReSyn, a large-scale IR dataset with balanced complexity, including real and synthetic images. We also established a unified training standard for IR models. Our RWKV-IR model integrates linear complexity RWKV into transformers for global and local receptive fields. It replaces Q-Shift with Depth-wise Convolution for local dependencies and combines Bi-directional attention for global-local awareness. The Cross-Bi-WKV module balances horizontal and vertical attention. Experiments show RWKV-IR’s effectiveness in image restoration.
Source link
lol
Exploring Real&Synthetic Dataset and Linear Attention in Image Restoration
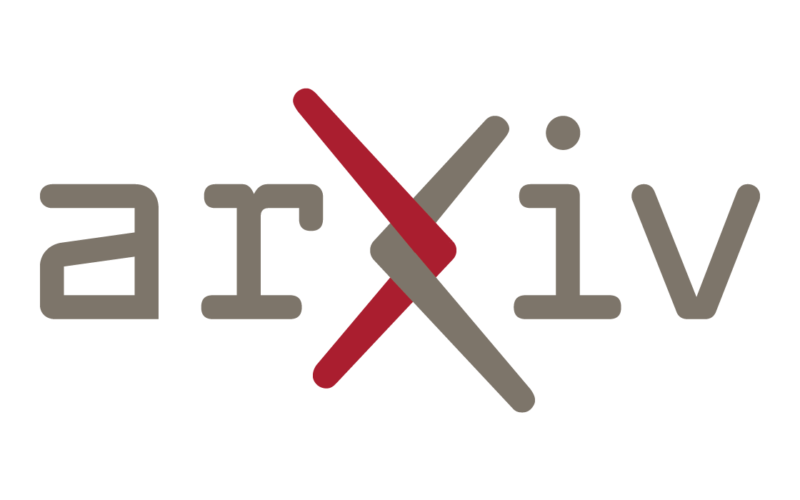