arXiv:2412.01958v1 Announce Type: new
Abstract: This paper evaluates the use of metamorphic relations to enhance the robustness and real-world performance of machine learning models. We propose a Metamorphic Retraining Framework, which applies metamorphic relations to data and utilizes semi-supervised learning algorithms in an iterative and adaptive multi-cycle process. The framework integrates multiple semi-supervised retraining algorithms, including FixMatch, FlexMatch, MixMatch, and FullMatch, to automate the retraining, evaluation, and testing of models with specified configurations. To assess the effectiveness of this approach, we conducted experiments on CIFAR-10, CIFAR-100, and MNIST datasets using a variety of image processing models, both pretrained and non-pretrained. Our results demonstrate the potential of metamorphic retraining to significantly improve model robustness as we show in our results that each model witnessed an increase of an additional flat 17 percent on average in our robustness metric.
Source link
lol
Enhancing Deep Learning Model Robustness through Metamorphic Re-Training
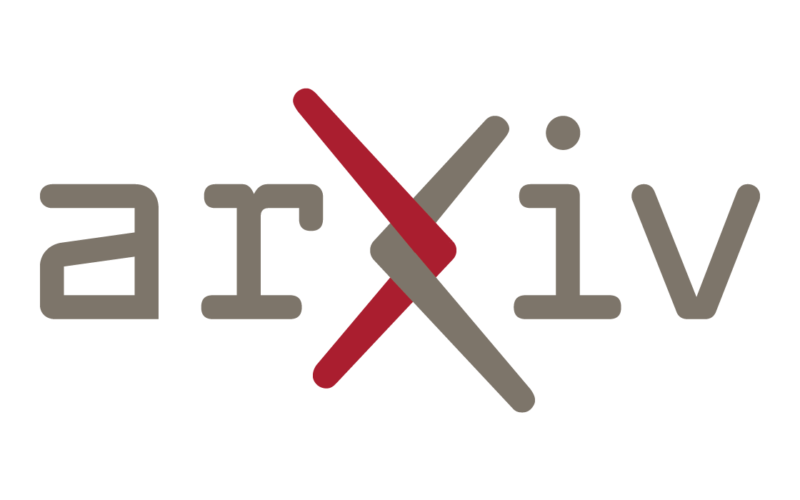