arXiv:2412.01926v1 Announce Type: new
Abstract: Several self-supervised learning (SSL) approaches have shown that redundancy reduction in the feature embedding space is an effective tool for representation learning. However, these methods consider a narrow notion of redundancy, focusing on pairwise correlations between features. To address this limitation, we formalize the notion of embedding space redundancy and introduce redundancy measures that capture more complex, higher-order dependencies. We mathematically analyze the relationships between these metrics, and empirically measure these redundancies in the embedding spaces of common SSL methods. Based on our findings, we propose Self Supervised Learning with Predictability Minimization (SSLPM) as a method for reducing redundancy in the embedding space. SSLPM combines an encoder network with a predictor engaging in a competitive game of reducing and exploiting dependencies respectively. We demonstrate that SSLPM is competitive with state-of-the-art methods and find that the best performing SSL methods exhibit low embedding space redundancy, suggesting that even methods without explicit redundancy reduction mechanisms perform redundancy reduction implicitly.
Source link
lol
Beyond Pairwise Correlations: Higher-Order Redundancies in Self-Supervised Representation Learning
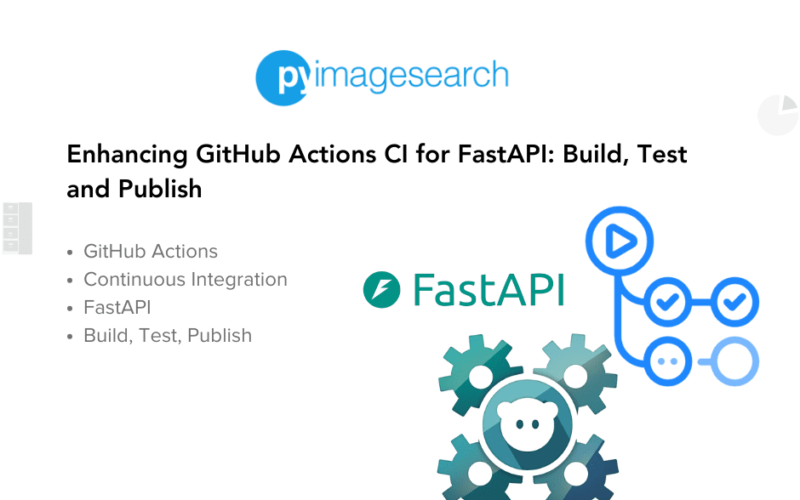