arXiv:2412.00323v1 Announce Type: new
Abstract: Large Language Models (LLMs) are trained on large corpora written by humans and demonstrate high performance on various tasks. However, as humans are susceptible to cognitive biases, which can result in irrational judgments, LLMs can also be influenced by these biases, leading to irrational decision-making. For example, changing the order of options in multiple-choice questions affects the performance of LLMs due to order bias. In our research, we first conducted an extensive survey of existing studies examining LLMs’ cognitive biases and their mitigation. The mitigation techniques in LLMs have the disadvantage that they are limited in the type of biases they can apply or require lengthy inputs or outputs. We then examined the effectiveness of two mitigation methods for humans, SoPro and AwaRe, when applied to LLMs, inspired by studies in crowdsourcing. To test the effectiveness of these methods, we conducted experiments on GPT-3.5 and GPT-4 to evaluate the influence of six biases on the outputs before and after applying these methods. The results demonstrate that while SoPro has little effect, AwaRe enables LLMs to mitigate the effect of these biases and make more rational responses.
Source link
lol
Cognitive Biases in Large Language Models: A Survey and Mitigation Experiments
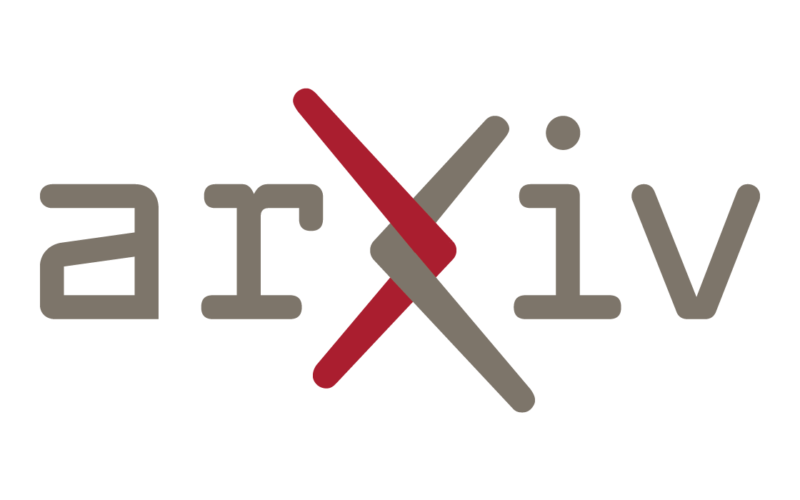