arXiv:2406.06607v1 Announce Type: new
Abstract: Fault detection is crucial in industrial systems to prevent failures and optimize performance by distinguishing abnormal from normal operating conditions. Data-driven methods have been gaining popularity for fault detection tasks as the amount of condition monitoring data from complex industrial systems increases. Despite these advances, early fault detection remains a challenge under real-world scenarios. The high variability of operating conditions and environments makes it difficult to collect comprehensive training datasets that can represent all possible operating conditions, especially in the early stages of system operation. Furthermore, these variations often evolve over time, potentially leading to entirely new data distributions in the future that were previously unseen. These challenges prevent direct knowledge transfer across different units and over time, leading to the distribution gap between training and testing data and inducing performance degradation of those methods in real-world scenarios. To overcome this, our work introduces a novel approach for continuous test-time domain adaptation. This enables early-stage robust anomaly detection by addressing domain shifts and limited data representativeness issues. We propose a Test-time domain Adaptation Anomaly Detection (TAAD) framework that separates input variables into system parameters and measurements, employing two domain adaptation modules to independently adapt to each input category. This method allows for effective adaptation to evolving operating conditions and is particularly beneficial in systems with scarce data. Our approach, tested on a real-world pump monitoring dataset, shows significant improvements over existing domain adaptation methods in fault detection, demonstrating enhanced accuracy and reliability.
Source link
lol
Continuous Test-time Domain Adaptation for Efficient Fault Detection under Evolving Operating Conditions
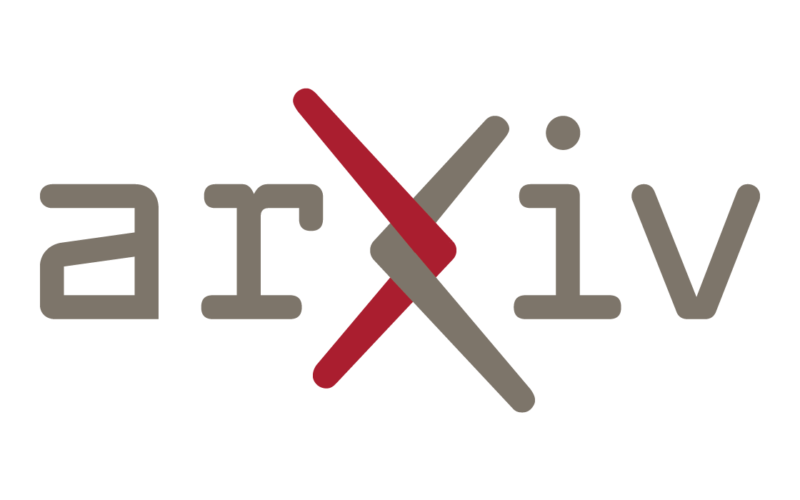