arXiv:2411.16775v1 Announce Type: new
Abstract: Instruction tuning has become an important step for finetuning pretrained language models to better follow human instructions and generalize on various tasks. Nowadays, pretrained language models become increasingly larger, and full parameter finetuning is overwhelmingly costly. Therefore, Parameter Efficient Finetuning (PEFT) has arisen as a cost-effective practice for instruction tuning because of significantly smaller computational, memory, and storage cost compared to full finetuning. Despite their widespread adaptations, the vast hyperparameter spaces, the number of PEFT methods, the different focus of instruction tuning capabilities make disentangling the impact of each aspect difficult. This study systematically investigates several representative PEFT methods, surveying the effect of hyperparameter choices including training hyperparameters and PEFT-specific hyperparameters, how different models sizes and the number of instruction tasks affect the performance, in-task-distribution memorization and open instruction following capability. Our empirical study shows that only LoRA and adapter can get close to full finetuning with ideal training settings. The ideal training setting includes an appropriate learning rate, largest LoRA rank or adapter size allowed and diverse training tasks. On the other hand, LoRA and adapter suffer from training instability if such an ideal training condition is not met. Additionally, LoRA requires a greater number of tasks for effective unseen task generalization, exhibit slower learning speed. Moreover, LoRA has weaker task-level memorization. Lastly, LoRA and adapter fall short in complex reasoning, coding and long-form generation compared to finetuning in open instruction tuning settings but it shows stronger capabilities compared to adapter.
Source link
lol
Parameter Efficient Instruction Tuning: An Empirical Study
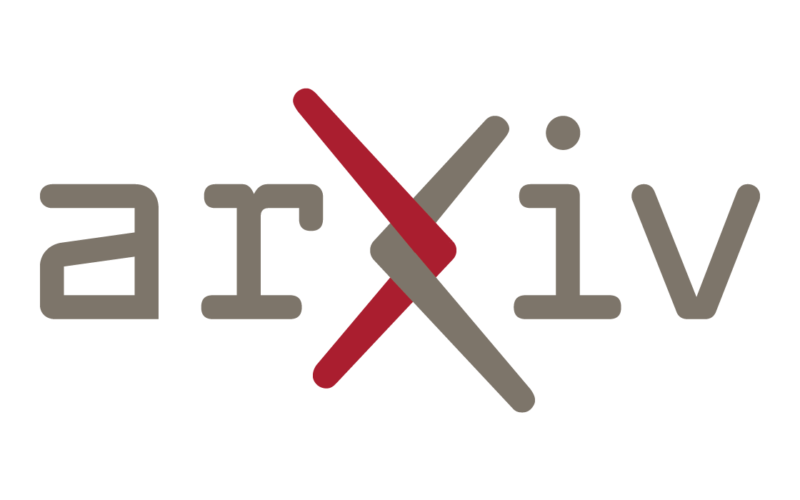