arXiv:2411.15244v1 Announce Type: new
Abstract: Large pre-trained Vision-Language Models (VLMs) such as Contrastive Language-Image Pre-Training (CLIP) have been shown to be susceptible to adversarial attacks, raising concerns about their deployment in safety-critical scenarios like autonomous driving and medical diagnosis. One promising approach for improving the robustness of pre-trained VLMs is Adversarial Prompt Tuning (APT), which combines adversarial training with prompt tuning. However, existing APT methods are mostly single-modal methods that design prompt(s) for only the visual or textual modality, limiting their effectiveness in either robustness or clean accuracy. In this work, we propose a novel method called Adversarial Prompt Distillation (APD) that combines APT with knowledge distillation to boost the adversarial robustness of CLIP. Specifically, APD is a bimodal method that adds prompts for both the visual and textual modalities while leveraging a cleanly pre-trained teacher CLIP model to distill and boost the performance of the student CLIP model on downstream tasks. Extensive experiments on multiple benchmark datasets demonstrate the superiority of our APD over the current state-of-the-art APT methods in terms of both natural and adversarial performances. The effectiveness of our APD method validates the possibility of using a non-robust teacher to improve the generalization and robustness of VLMs.
Source link
lol
Adversarial Prompt Distillation for Vision-Language Models
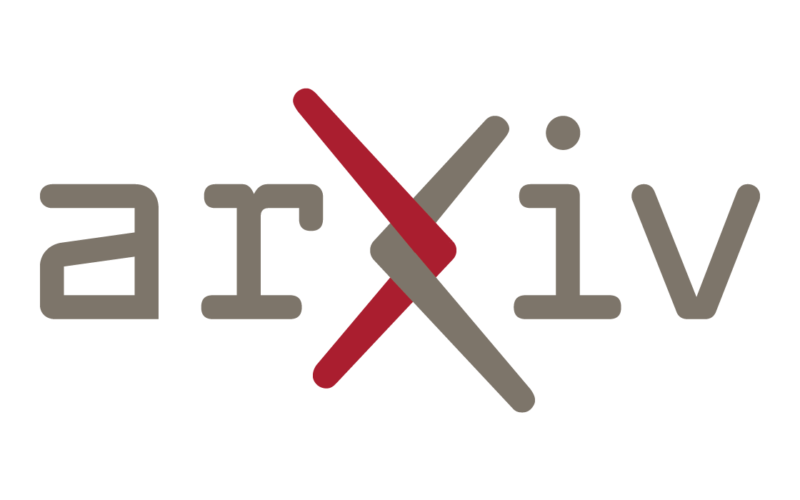