arXiv:2411.15287v1 Announce Type: new
Abstract: Large language models (LLMs) have demonstrated remarkable capabilities across a wide range of natural language processing tasks. However, their tendency to exhibit sycophantic behavior – excessively agreeing with or flattering users – poses significant risks to their reliability and ethical deployment. This paper provides a technical survey of sycophancy in LLMs, analyzing its causes, impacts, and potential mitigation strategies. We review recent work on measuring and quantifying sycophantic tendencies, examine the relationship between sycophancy and other challenges like hallucination and bias, and evaluate promising techniques for reducing sycophancy while maintaining model performance. Key approaches explored include improved training data, novel fine-tuning methods, post-deployment control mechanisms, and decoding strategies. We also discuss the broader implications of sycophancy for AI alignment and propose directions for future research. Our analysis suggests that mitigating sycophancy is crucial for developing more robust, reliable, and ethically-aligned language models.
Source link
lol
Sycophancy in Large Language Models: Causes and Mitigations
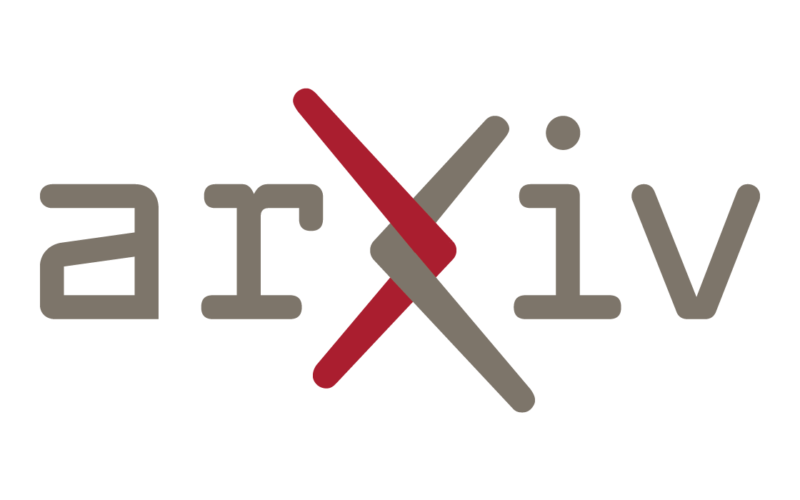