arXiv:2411.13691v1 Announce Type: new
Abstract: We designed a Retrieval-Augmented Generation (RAG) system to provide large language models with relevant documents for answering domain-specific questions about Pittsburgh and Carnegie Mellon University (CMU). We extracted over 1,800 subpages using a greedy scraping strategy and employed a hybrid annotation process, combining manual and Mistral-generated question-answer pairs, achieving an inter-annotator agreement (IAA) score of 0.7625. Our RAG framework integrates BM25 and FAISS retrievers, enhanced with a reranker for improved document retrieval accuracy. Experimental results show that the RAG system significantly outperforms a non-RAG baseline, particularly in time-sensitive and complex queries, with an F1 score improvement from 5.45% to 42.21% and recall of 56.18%. This study demonstrates the potential of RAG systems in enhancing answer precision and relevance, while identifying areas for further optimization in document retrieval and model training.
Source link
lol
Retrieval-Augmented Generation for Domain-Specific Question Answering: A Case Study on Pittsburgh and CMU
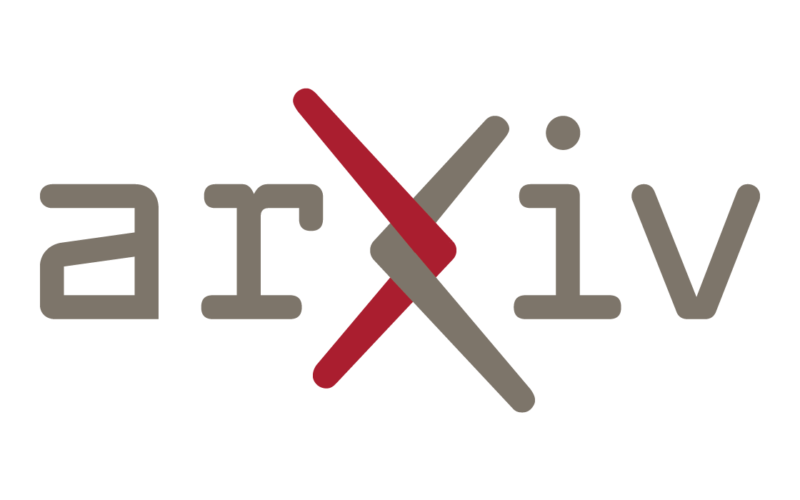