Generative AI fever shows no signs of cooling off. As pressure and excitement build to execute strong GenAI strategies, data leaders and practitioners are searching for the best platform, tools and use cases to help them get there.
How is this playing out in the real world? We just launched the 2024 State of Data + AI, which leverages data from our 10,000 global customers, to understand how organizations across industries are approaching AI. While our report covers a broad range of themes relevant to any data-driven company, clear trends emerged on the GenAI journey.
Here’s a snapshot of what we discovered:
Top 10 data and AI products: the GenAI Stack is forming
With any new technology, developers will experiment with lots of different tools to figure out what works best for them.
Our Top 10 Data and AI Products showcase the most widely-adopted integrations on the Databricks Data Intelligence Platform. From data integration to model development, this list shows how companies are investing in their stack to support new GenAI priorities:
Hugging Face transformers jump to No. 2
In just 12 months, Hugging Face jumps from spot #4 to spot #2. Many companies use the open source platform’s transformer models together with their enterprise data to build and fine-tune foundation models.
LangChain becomes top product months after integration
LangChain, an open source toolchain for working with and building proprietary LLMs, rose to spot #4 in less than one year of integration. When companies build their own modern LLM applications and work with specialized transformer-related Python libraries to train the models, LangChain enables them to develop prompt interfaces or integrations to other systems.
Enterprise GenAI is all about customizing LLMs
Last year, our data showed SaaS LLMs as the “it” tool of 2023, when analyzing the most popular LLM Python libraries. This year, our data is showing the use of general- purpose LLMs continue but with slowed year-over-year growth.
This year’s strategy has taken a major shift. Our data shows that companies are hyper-focused on augmenting LLMs with their custom data vs just using standalone off-the-shelf LLMs.
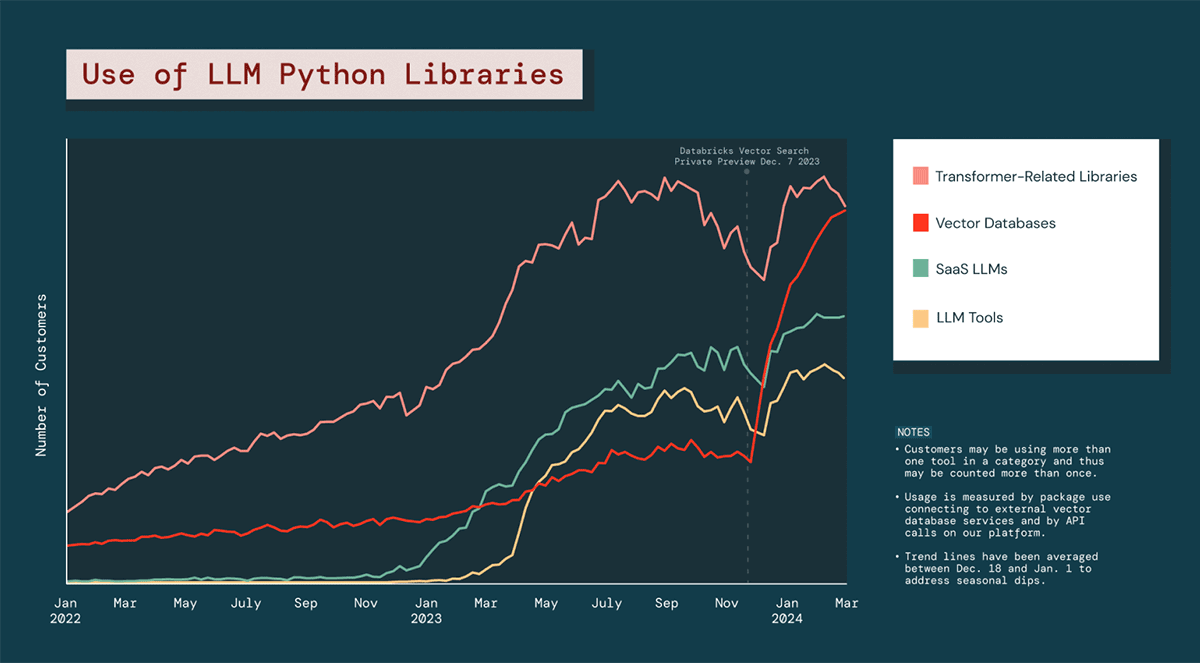
Businesses want to harness the power of SaaS LLMs, but also improve the accuracy and mold the underlying models to better work for them. With RAG, companies can use something like an employee handbook or their own financial statements so the model can start to generate outputs that are specific to the business. And there’s huge demand across our customers to build these customized systems. The use of vector databases, a vital component of RAG models, grew 377% in the last year – including a 186% jump after Databricks Vector Search went into Public Preview.
The enterprise AI strategy and open LLMs
As companies build their technology stacks, open source is making its mark. In fact, 9 of the 10 top products are open source, including our two big GenAI players: Hugging Face and LangChain.
Open-source LLMs also offer many enterprise benefits, such as the ability to customize them to your organization’s unique needs and use cases. We analyzed the open source model usage of Meta Llama and Mistral, the two biggest players, to understand which models companies gravitated toward.
With each model, there is a trade-off between cost, latency and performance. Together, usage of the two smallest Meta Llama 2 models (7B and 13B) is significantly higher than the largest, Meta Llama 2 70B.
Across both Llama and Mistral users, 77% choose models with 13B parameters or fewer. This suggests that companies care significantly about cost and latency.
Dive deeper into these and other trends in the 2024 State of Data + AI. Consider it your playbook for an effective data and AI strategy. Download the full report here.
Source link
lol