arXiv:2411.10666v1 Announce Type: new
Abstract: Large Language Models (LLMs) have revolutionized natural language processing by unifying tasks into text generation, yet their large parameter sizes and autoregressive nature limit inference speed. SAM-Decoding addresses this by introducing a novel retrieval-based speculative decoding method that uses a suffix automaton for efficient and accurate draft generation. Unlike n-gram matching used by the existing method, SAM-Decoding finds the longest suffix match in generating text and text corpuss, achieving an average time complexity of $O(1)$ per generation step. SAM-Decoding constructs static and dynamic suffix automatons for the text corpus and input prompts, respectively, enabling fast and precise draft generation. Meanwhile, it is designed as an approach that can be combined with existing methods, allowing SAM-Decoding to adaptively select a draft generation strategy based on the matching length, thus increasing the inference speed of the LLM. When combined with Token Recycling, evaluations show SAM-Decoding outperforms existing model-free methods, achieving a speedup of $2.27times$ over autoregressive decoding on Spec-Bench. When combined with EAGLE2, it reaches a speedup of $2.49times$, surpassing all current approaches. Our code is available at https://github.com/hyx1999/SAM-Decoding.
Source link
lol
SAM Decoding: Speculative Decoding via Suffix Automaton
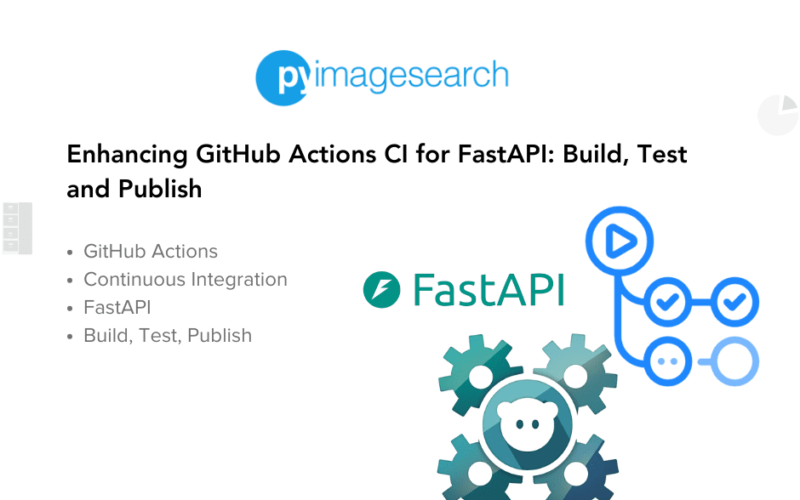