arXiv:2411.09686v1 Announce Type: cross
Abstract: Several statistical models for regression of a function $F$ on $mathbb{R}^d$ without the statistical and computational curse of dimensionality exist, for example by imposing and exploiting geometric assumptions on the distribution of the data (e.g. that its support is low-dimensional), or strong smoothness assumptions on $F$, or a special structure $F$. Among the latter, compositional models assume $F=fcirc g$ with $g$ mapping to $mathbb{R}^r$ with $rll d$, have been studied, and include classical single- and multi-index models and recent works on neural networks. While the case where $g$ is linear is rather well-understood, much less is known when $g$ is nonlinear, and in particular for which $g$’s the curse of dimensionality in estimating $F$, or both $f$ and $g$, may be circumvented. In this paper, we consider a model $F(X):=f(Pi_gamma X) $ where $Pi_gamma:mathbb{R}^dto[0,rm{len}_gamma]$ is the closest-point projection onto the parameter of a regular curve $gamma: [0,rm{len}_gamma]tomathbb{R}^d$ and $f:[0,rm{len}_gamma]tomathbb{R}^1$. The input data $X$ is not low-dimensional, far from $gamma$, conditioned on $Pi_gamma(X)$ being well-defined. The distribution of the data, $gamma$ and $f$ are unknown. This model is a natural nonlinear generalization of the single-index model, which corresponds to $gamma$ being a line. We propose a nonparametric estimator, based on conditional regression, and show that under suitable assumptions, the strongest of which being that $f$ is coarsely monotone, it can achieve the $one$-$dimensional$ optimal min-max rate for non-parametric regression, up to the level of noise in the observations, and be constructed in time $mathcal{O}(d^2nlog n)$. All the constants in the learning bounds, in the minimal number of samples required for our bounds to hold, and in the computational complexity are at most low-order polynomials in $d$.
Source link
lol
Conditional regression for the Nonlinear Single-Variable Model
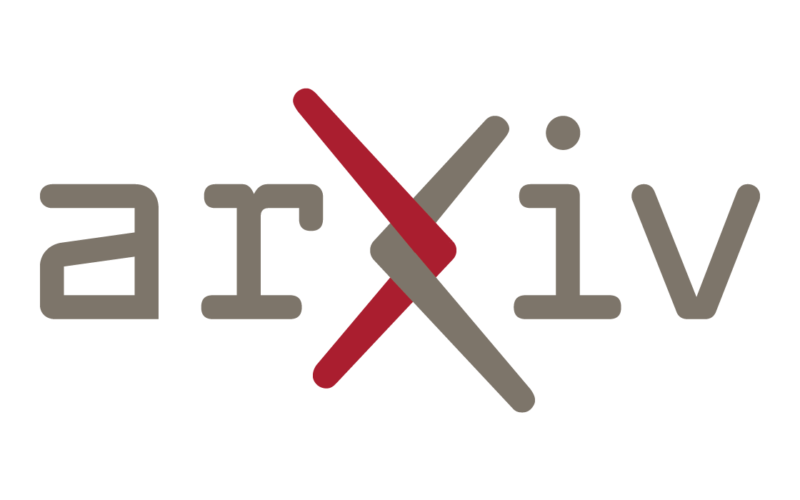