arXiv:2411.08861v1 Announce Type: cross
Abstract: Relationships of cause and effect are of prime importance for explaining scientific phenomena. Often, rather than just understanding the effects of causes, researchers also wish to understand how a cause $X$ affects an outcome $Y$ mechanistically — i.e., what are the causal pathways that are activated between $X$ and $Y$. For analyzing such questions, a range of methods has been developed over decades under the rubric of causal mediation analysis. Traditional mediation analysis focuses on decomposing the average treatment effect (ATE) into direct and indirect effects, and therefore focuses on the ATE as the central quantity. This corresponds to providing explanations for associations in the interventional regime, such as when the treatment $X$ is randomized. Commonly, however, it is of interest to explain associations in the observational regime, and not just in the interventional regime. In this paper, we introduce text{variation analysis}, an extension of mediation analysis that focuses on the total variation (TV) measure between $X$ and $Y$, written as $mathrm{E}[Y mid X=x_1] – mathrm{E}[Y mid X=x_0]$. The TV measure encompasses both causal and confounded effects, as opposed to the ATE which only encompasses causal (direct and mediated) variations. In this way, the TV measure is suitable for providing explanations in the natural regime and answering questions such as “why is $X$ associated with $Y$?”. Our focus is on decomposing the TV measure, in a way that explicitly includes direct, indirect, and confounded variations. Furthermore, we also decompose the TV measure to include interaction terms between these different pathways. Subsequently, interaction testing is introduced, involving hypothesis tests to determine if interaction terms are significantly different from zero. If interactions are not significant, more parsimonious decompositions of the TV measure can be used.
Source link
lol
Interaction Testing in Variation Analysis
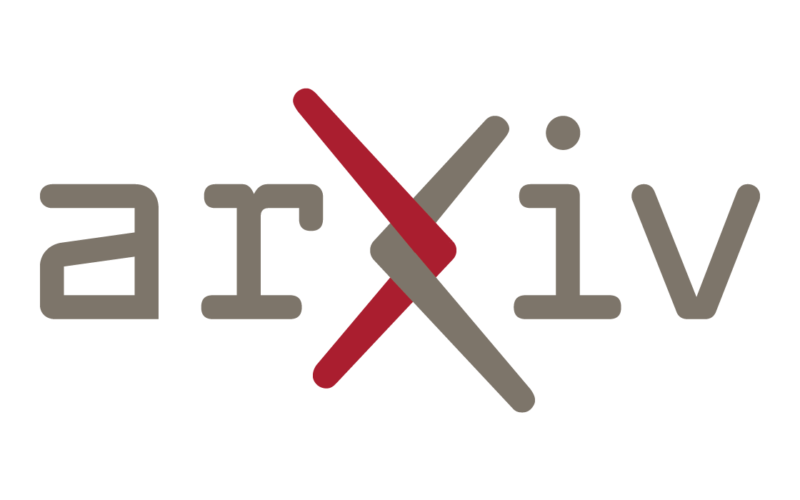