arXiv:2411.08249v1 Announce Type: new
Abstract: Retrieval-augmented generation (RAG) is a central component of modern LLM systems, particularly in scenarios where up-to-date information is crucial for accurately responding to user queries or when queries exceed the scope of the training data. The advent of time-series foundation models (TSFM), such as Chronos, and the need for effective zero-shot forecasting performance across various time-series domains motivates the question: Do benefits of RAG similarly carry over to time series forecasting? In this paper, we advocate that the dynamic and event-driven nature of time-series data makes RAG a crucial component of TSFMs and introduce a principled RAG framework for time-series forecasting, called Retrieval Augmented Forecasting (RAF). Within RAF, we develop efficient strategies for retrieving related time-series examples and incorporating them into forecast. Through experiments and mechanistic studies, we demonstrate that RAF indeed improves the forecasting accuracy across diverse time series domains and the improvement is more significant for larger TSFM sizes.
Source link
lol
Retrieval Augmented Time Series Forecasting
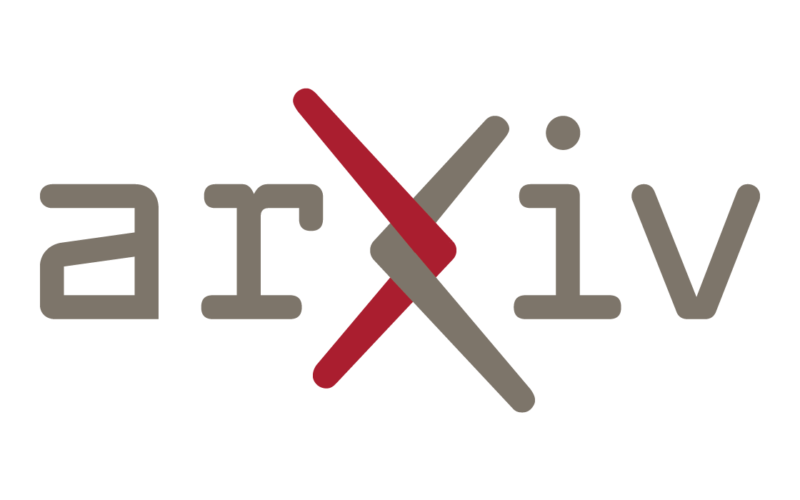